AI and Machine Learning in Clinical Trials
Clinical trials are the cornerstone of medical research, playing a crucial role in the development of new treatments and therapies. However, these trials are often fraught with challenges such as high costs, lengthy durations, and difficulties in patient recruitment and retention. As the demand for more efficient and effective clinical trials grows, the healthcare industry is increasingly turning to artificial intelligence (AI) and machine learning (ML) to revolutionize the way these studies are conducted.
AI and ML have already made significant strides in various sectors, and their application in clinical trials holds immense potential. By harnessing the power of these advanced technologies, we can optimize clinical trial processes, enhance patient outcomes, and ultimately bring new treatments to market more swiftly and safely. This blog explores the transformative role of AI and ML in clinical trials, examining how they can address the challenges of traditional methods and pave the way for a new era of medical research.
Join us as we delve into the intricacies of AI and machine learning, uncovering their applications in patient recruitment, trial design, data management, and outcome prediction. We will also highlight real-world examples of successful AI implementations in clinical trials, discuss the ethical considerations and challenges, and look ahead to the future prospects of these groundbreaking technologies. Whether you are a healthcare professional, researcher, or simply curious about the advancements in medical science, this exploration into AI and machine learning in clinical trials will offer valuable insights and inspire a deeper understanding of the future of healthcare.
Overview of Clinical Trials
Clinical trials are systematic investigations in human participants designed to evaluate the efficacy and safety of new medical interventions, including drugs, devices, and treatment protocols. These trials are essential for advancing medical knowledge and improving patient care, ensuring that new treatments are both effective and safe before they become widely available. The process of clinical trials is typically divided into four main phases:
Phase I: Safety and Dosage
The primary focus of Phase I trials is to evaluate the safety of a new intervention. This initial phase involves a small group of healthy volunteers or patients and aims to determine the appropriate dosage range and identify any potential side effects. Researchers closely monitor participants to understand how the intervention is metabolized and excreted by the body.
Phase II: Efficacy and Side Effects
Phase II trials involve a larger group of participants and aim to assess the efficacy of the intervention. This phase provides preliminary data on whether the treatment works as intended for a specific condition or disease. Additionally, researchers continue to monitor for side effects and further refine dosage levels based on the data collected.
Phase III: Confirmation and Monitoring
In Phase III trials, the intervention is tested on an even larger group of participants to confirm its efficacy and monitor adverse reactions in a more diverse population. This phase often involves randomized and blinded trials to eliminate bias and ensure robust data. Successful Phase III trials are critical for obtaining regulatory approval from bodies such as the FDA or EMA.
Phase IV: Post-marketing Studies
After an intervention has been approved and is on the market, Phase IV trials, also known as post-marketing surveillance, are conducted to gather additional information on the treatment's long-term effectiveness and safety. These studies can identify rare side effects, assess the intervention's performance in different populations, and provide insights into its optimal use in clinical practice.
Challenges Faced in Traditional Clinical Trials
Despite their importance, traditional clinical trials face several significant challenges that can hinder their efficiency and effectiveness:
High Costs
Conducting clinical trials is an expensive endeavor, with costs often reaching millions of dollars. Expenses include recruiting and compensating participants, paying for staff and facilities, and covering the costs of data collection and analysis. These high costs can be a barrier to innovation, particularly for smaller organizations.
Long Duration
Clinical trials are notoriously time-consuming, often taking several years to complete. The lengthy process of recruiting participants, collecting data, and analyzing results can delay the availability of new treatments, potentially affecting patient outcomes and healthcare costs.
Patient Recruitment and Retention
Finding and retaining participants for clinical trials is one of the most challenging aspects of the process. Many trials struggle to meet their recruitment targets, and even when participants are enrolled, maintaining their engagement throughout the trial can be difficult. This can lead to delays and incomplete data, compromising the trial's validity.
Data Management
Managing the vast amounts of data generated during clinical trials is a complex task. Ensuring data accuracy, consistency, and security requires significant resources and sophisticated systems. Traditional methods of data management can be prone to errors and inefficiencies, further complicating the trial process.
The Role of AI and Machine Learning
Given these challenges, the integration of AI and machine learning into clinical trials offers promising solutions. These technologies can enhance various aspects of the trial process, from patient recruitment to data analysis, ultimately leading to more efficient, cost-effective, and successful outcomes. In the following sections, we will explore how AI and machine learning are transforming clinical trials, optimizing processes, and improving results for patients and researchers alike.
The Role of AI and Machine Learning
Artificial Intelligence (AI) and Machine Learning (ML) are transforming numerous industries, and clinical trials are no exception. By leveraging the power of these advanced technologies, researchers can optimize the design and execution of clinical trials, addressing many of the challenges that have traditionally impeded progress. AI and ML can be applied at various stages of the clinical trial process, providing insights and efficiencies that were previously unattainable.
Predictive Analytics
AI and ML algorithms excel at predictive analytics, enabling researchers to forecast outcomes and trends based on vast datasets. In clinical trials, predictive analytics can be used to:
- Identify patient populations that are most likely to benefit from a new treatment, ensuring trials are targeted and efficient.
- Forecast trial timelines and costs, allowing for better planning and resource allocation.
- Predict patient responses and potential side effects, which can improve patient safety and trial outcomes.
Natural Language Processing
Natural Language Processing (NLP) is a subset of AI that focuses on the interaction between computers and human language. NLP can analyze large volumes of unstructured data, such as medical records and scientific literature, to extract valuable information. In clinical trials, NLP can be used to:
- Screen and recruit patients by analyzing electronic health records (EHRs) and identifying eligible candidates.
- Analyze clinical trial protocols and documents, ensuring compliance with regulatory requirements and identifying potential issues early.
- Monitor and analyze patient-reported outcomes, providing real-time insights into patient experiences and treatment efficacy.
Image Analysis
AI-driven image analysis can significantly enhance the accuracy and efficiency of interpreting medical images, such as MRI scans, X-rays, and histopathology slides. In clinical trials, image analysis can be used to:
- Automate the detection and quantification of disease markers, reducing the time and variability associated with manual analysis.
- Track disease progression and treatment responses with greater precision.
- Enhance the consistency and reproducibility of imaging data, ensuring reliable trial results.
Optimizing Clinical Trial Processes with AI
Patient Recruitment and Retention:
One of the most significant challenges in clinical trials is recruiting and retaining participants. AI and ML can streamline this process by:
- Identifying eligible patients through EHRs and other data sources, reducing the time and effort required for recruitment.
- Personalizing patient engagement using AI-driven apps and platforms that provide tailored information and support, improving retention rates.
- Predicting dropout risks and intervening proactively to address potential issues, ensuring that trials are completed successfully.
Trial Design and Planning
AI and ML can enhance trial design and planning by:
- Using predictive modeling to identify the most effective trial designs, reducing the likelihood of costly and time-consuming protocol amendments.
- Implementing adaptive trial designs that allow for modifications based on real-time data analysis, improving efficiency and patient safety.
- Optimizing resource allocation by forecasting recruitment rates, dropout rates, and other critical factors, ensuring that trials are conducted as efficiently as possible.
Data Management and Analysis
Managing and analyzing the vast amounts of data generated during clinical trials can be daunting. AI and ML can streamline these tasks by:
- Automating data collection and entry, reducing the risk of errors and freeing up valuable time for researchers.
- Ensuring data integrity and consistency through advanced validation algorithms, enhancing the reliability of trial results.
- Applying advanced analytics to uncover insights and patterns in the data, enabling faster and more accurate decision-making.
Improving Outcomes with Machine Learning
Precision Medicine
Machine learning plays a crucial role in the development of precision medicine, which aims to tailor treatments to individual patients based on their unique characteristics. In clinical trials, ML can be used to:
- Develop personalized treatment plans by analyzing patient data, including genetic information, lifestyle factors, and clinical history.
- Predict patient responses to treatments, allowing for more targeted and effective interventions.
- Identify biomarkers that can serve as indicators of treatment efficacy, enabling more precise and informed decision-making.
Safety Monitoring
Ensuring patient safety is paramount in clinical trials. AI and ML can enhance safety monitoring by:
- Detecting adverse events early through continuous monitoring and real-time data analysis, allowing for prompt intervention.
- Predicting potential safety issues based on historical data and patient characteristics, enabling proactive risk management.
- Enhancing pharmacovigilance by automating the detection and reporting of safety signals, ensuring regulatory compliance and patient protection.
Regulatory Compliance
Navigating the complex regulatory landscape is a critical aspect of conducting clinical trials. AI and ML can support regulatory compliance by:
- Automating reporting and documentation, reducing the administrative burden on researchers and ensuring timely submissions.
- Ensuring trials meet regulatory requirements through advanced validation algorithms and real-time monitoring.
- Facilitating communication with regulatory bodies by providing detailed, data-driven insights and reports.
In the next sections, we will explore real-world examples of AI and machine learning in clinical trials, discuss the challenges and ethical considerations associated with these technologies, and look ahead to the future prospects of AI-driven clinical trials.
Optimizing Clinical Trial Processes with AI
Artificial intelligence (AI) is revolutionizing the landscape of clinical trials by enhancing efficiency, reducing costs, and improving outcomes. Here, we delve into how AI optimizes various aspects of clinical trial processes, from patient recruitment to data management and analysis.
Patient Recruitment and Retention
One of the most critical and challenging aspects of clinical trials is recruiting and retaining participants. AI can significantly streamline these processes:
- Identifying Eligible Patients: AI algorithms can sift through vast amounts of electronic health records (EHRs) to identify patients who meet the specific criteria for a trial. This reduces the time and resources spent on manual screening and ensures a more targeted approach to recruitment.
- Enhancing Patient Engagement: AI-driven platforms and applications can provide personalized communication and support to participants, keeping them informed and engaged throughout the trial. These tools can send reminders for appointments, medication adherence, and follow-up surveys, improving retention rates.
- Predicting Dropout Risks: AI can analyze patient behavior and demographic data to predict which participants are at risk of dropping out. By identifying these risks early, researchers can intervene with targeted strategies to keep participants engaged, such as providing additional support or addressing specific concerns.
Trial Design and Planning
AI can also play a pivotal role in designing and planning clinical trials, making them more efficient and effective:
- Predictive Modeling for Trial Design: AI can use historical data and predictive modeling to identify the most effective trial designs. This helps in selecting appropriate endpoints, sample sizes, and methodologies, reducing the likelihood of protocol amendments and associated costs.
- Adaptive Trial Designs: AI enables the implementation of adaptive trial designs, which allow modifications to the trial protocol based on interim results. This flexibility can lead to faster and more accurate identification of effective treatments while ensuring patient safety.
- Resource Allocation Optimization: AI can forecast recruitment rates, dropout rates, and other critical factors, helping researchers allocate resources more effectively. This ensures that trials are conducted efficiently, with minimal waste of time and money.
Data Management and Analysis
The management and analysis of data are central to the success of clinical trials. AI enhances these processes in several ways:
- Automating Data Collection and Entry: AI-powered tools can automate the collection and entry of data from various sources, such as patient-reported outcomes, wearables, and EHRs. This reduces the risk of errors and frees up valuable time for researchers to focus on analysis and decision-making.
- Ensuring Data Integrity and Consistency: Advanced AI algorithms can validate data in real time, ensuring accuracy and consistency across different sources. This enhances the reliability of trial results and facilitates regulatory compliance.
- Advanced Analytics for Insights: AI can analyze complex datasets to uncover patterns and insights that may not be immediately apparent through traditional methods. This enables faster and more accurate decision-making, leading to improved trial outcomes.
By optimizing patient recruitment, trial design, data management, and analysis, AI is transforming clinical trials into more efficient, cost-effective, and successful endeavors. In the next section, we will explore how machine learning improves clinical trial outcomes, focusing on precision medicine, safety monitoring, and regulatory compliance.
Improving Outcomes with Machine Learning
Machine Learning (ML), a specialized branch of artificial intelligence, excels in processing vast and complex datasets, identifying patterns, and making data-driven predictions. In clinical trials, ML is pivotal in enhancing outcomes through precision medicine, advanced safety monitoring, and improved regulatory compliance. Here's how ML is transforming these critical areas:
Precision Medicine
Precision medicine aims to customize healthcare, tailoring medical treatment to the individual characteristics of each patient. ML is a crucial enabler of this approach:
- Personalized Treatment Plans: ML algorithms can analyze diverse data sources, including genetic information, lifestyle factors, and medical history, to create highly personalized treatment plans. This individualized approach ensures that patients receive the most effective therapies based on their unique profiles, leading to better outcomes and fewer adverse reactions.
- Predicting Patient Responses: By leveraging historical data from previous trials and patient outcomes, ML models can predict how individual patients are likely to respond to a particular treatment. This predictive capability helps in selecting the most suitable candidates for trials and in optimizing treatment protocols, ultimately improving efficacy and safety.
- Identifying Biomarkers: ML can identify biomarkers—biological indicators that signal disease progression or treatment response. These biomarkers are invaluable for stratifying patients and tailoring interventions, enhancing the precision and effectiveness of clinical trials.
Safety Monitoring
Ensuring patient safety is a primary concern in clinical trials. ML significantly enhances safety monitoring by providing real-time insights and predictive capabilities:
- Early Detection of Adverse Events: ML algorithms can continuously monitor patient data for signs of adverse events, detecting issues much earlier than traditional methods. This early detection allows for prompt intervention, minimizing risks and enhancing patient safety.
- Predictive Safety Models: By analyzing data from past trials, ML can identify patterns that precede adverse events. These predictive models help in assessing the risk profiles of new treatments, enabling proactive safety measures and informed decision-making.
- Enhanced Pharmacovigilance: ML automates the process of pharmacovigilance—the monitoring of drug safety post-market. By rapidly identifying and analyzing safety signals, ML helps maintain high safety standards and ensures compliance with regulatory requirements.
Regulatory Compliance
Navigating the regulatory landscape is complex and time-consuming. ML aids in ensuring compliance and streamlining interactions with regulatory bodies:
- Automating Reporting and Documentation: ML can automate the generation of reports and documentation required for regulatory submissions. This automation reduces administrative burdens, ensures accuracy, and speeds up the submission process.
- Ensuring Trials Meet Regulatory Standards: ML continuously monitors trial data to ensure adherence to regulatory standards, including protocol compliance, informed consent processes, and data integrity. This real-time oversight helps avoid deviations that could compromise the trial.
- Facilitating Communication with Regulatory Bodies: ML tools generate detailed, data-driven insights and reports that facilitate transparent and effective communication with regulatory authorities. This clarity and thoroughness help build trust and ensure that trials meet all necessary regulatory criteria.
Case Studies and Real-World Examples
To illustrate the transformative impact of AI and ML in clinical trials, here are some real-world examples:
- Pharmaceutical Companies: Leading pharmaceutical companies are integrating AI and ML into their clinical trial processes to enhance efficiency and outcomes. For example, AI-driven platforms are being used to identify eligible patients quickly, predict trial timelines accurately, and monitor safety more effectively, resulting in faster and more cost-effective trials.
- Tech and Healthcare Collaborations: Collaborations between tech companies and healthcare providers have led to innovative solutions in clinical trials. For instance, partnerships focusing on AI-driven image analysis have significantly improved the accuracy of disease diagnosis and treatment monitoring, demonstrating the potential of AI to enhance clinical outcomes.
Noteworthy Example: Notable Labs

Notable Labs leverages AI and machine learning to enhance clinical trials through its Predictive Precision Medicine Platform (PPMP). This platform integrates biology, engineering, and data science to analyze complex bio-assay conditions and computational algorithms. By generating a massive data repository with 190 billion lines of data from thousands of patient samples and therapeutic compounds, Notable Labs can identify patients who will respond to treatments with 97% accuracy. This approach not only optimizes response predictions but also accelerates the development of promising therapeutic assets.
These case studies highlight the tangible benefits of AI and ML in optimizing clinical trials, from improving patient recruitment to ensuring safety and regulatory compliance.
Challenges and Ethical Considerations
While AI and ML offer numerous benefits in clinical trials, they also present challenges and ethical considerations that need careful attention:
- Data Privacy and Security: Protecting patient data privacy and security is paramount. Robust measures must be implemented to safeguard sensitive information and ensure compliance with data protection regulations.
- Ethical Issues in AI Decision-Making: The use of AI in decision-making raises ethical questions, particularly regarding transparency, accountability, and bias. It is crucial to ensure that AI algorithms are transparent, unbiased, and ethically sound to maintain trust and integrity in clinical trials.
- Regulatory Hurdles: The regulatory landscape for AI and ML in clinical trials is still evolving. Establishing standardized guidelines and ensuring regulatory alignment is critical for the widespread adoption of these technologies. Collaborative efforts between regulatory bodies, AI developers, and healthcare providers are necessary to address these challenges.
Future Prospects
The future of AI and ML in clinical trials looks promising, with potential advancements and innovations on the horizon:
- Evolving AI Technologies: As AI technologies continue to evolve, we can expect more sophisticated tools for optimizing clinical trials. This includes improved predictive models, more efficient data analysis techniques, and enhanced patient engagement platforms, leading to even more effective and streamlined trials.
- Increased Collaboration: Collaboration between AI developers, healthcare providers, and regulatory bodies will be crucial for driving innovation and ensuring the successful implementation of AI in clinical trials. These partnerships can help overcome challenges, address ethical considerations, and promote the broader adoption of AI and ML.
- Broader Adoption: As the benefits of AI and ML become more evident, we can anticipate wider adoption of these technologies across the healthcare industry. This will lead to more efficient clinical trials, better patient outcomes, and a faster pace of medical innovation, ultimately transforming the landscape of clinical research.
Conclusion
AI and machine learning are transforming clinical trials, offering solutions to longstanding challenges and paving the way for a new era of medical research. By optimizing patient recruitment, trial design, data management, and outcome prediction, these technologies enhance the efficiency, cost-effectiveness, and success of clinical trials. As we continue to explore and develop AI-driven solutions, the future of clinical trials looks brighter, promising improved patient outcomes and accelerated medical advancements.
To stay informed about the latest advancements in AI and machine learning in healthcare, subscribe to our blog and join the conversation. If you are a healthcare professional or researcher, explore AI solutions to enhance your clinical trials and collaborate with tech companies to drive innovation. Together, we can revolutionize clinical trials and improve patient care.
By embracing the transformative potential of AI and ML, we can overcome the challenges of traditional clinical trials and usher in a new era of medical research and patient care.
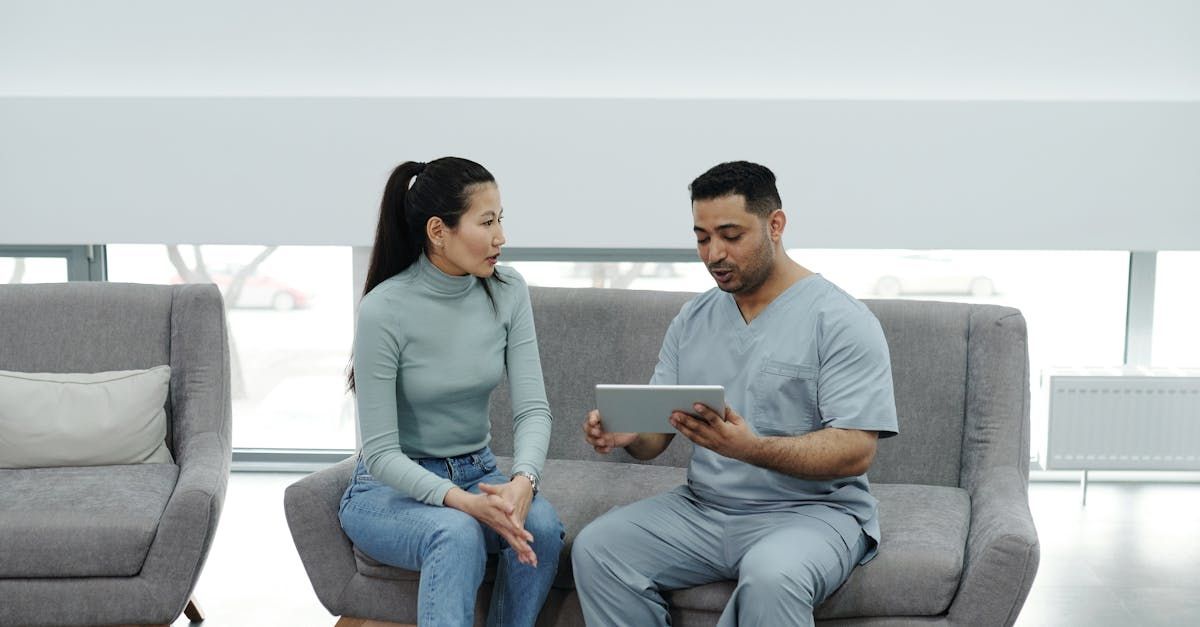
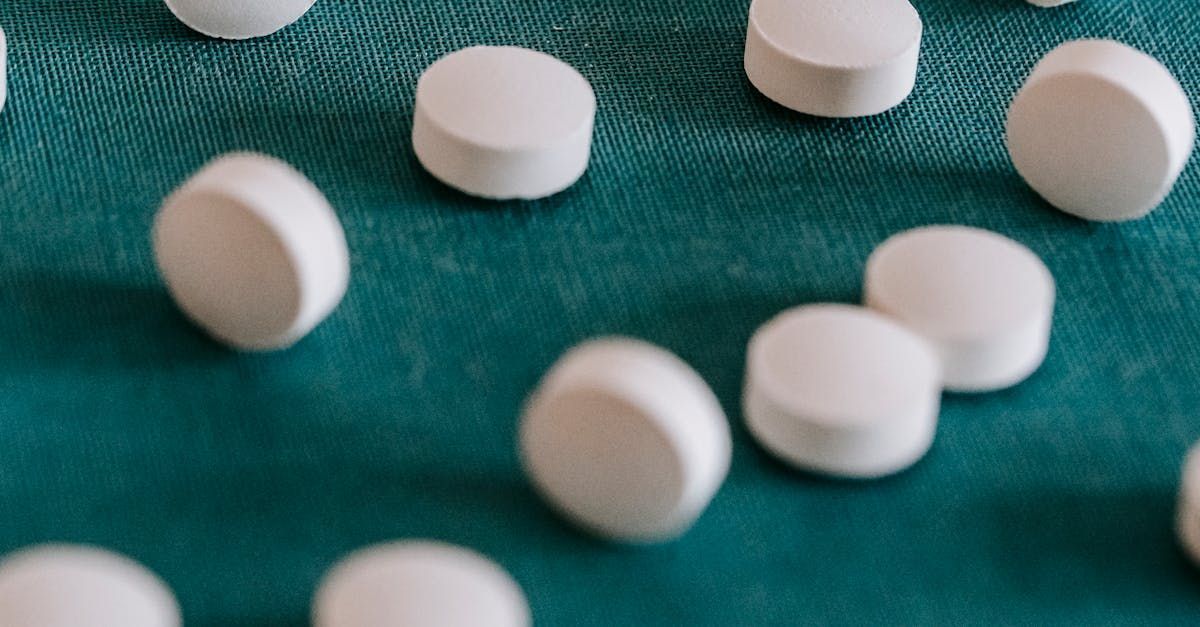
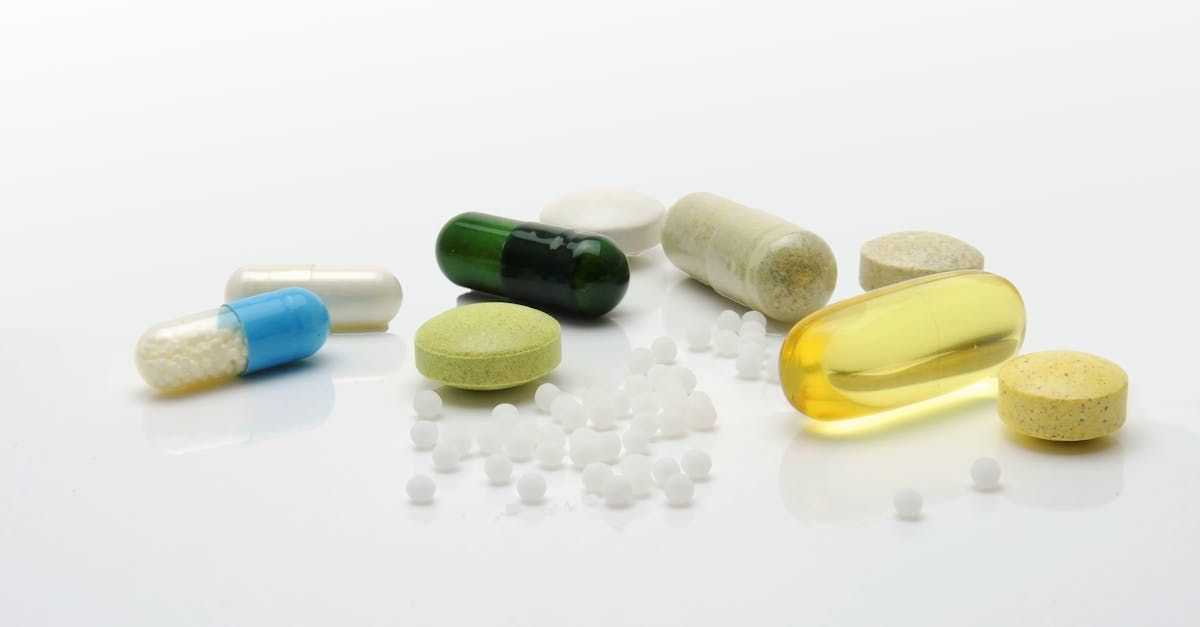
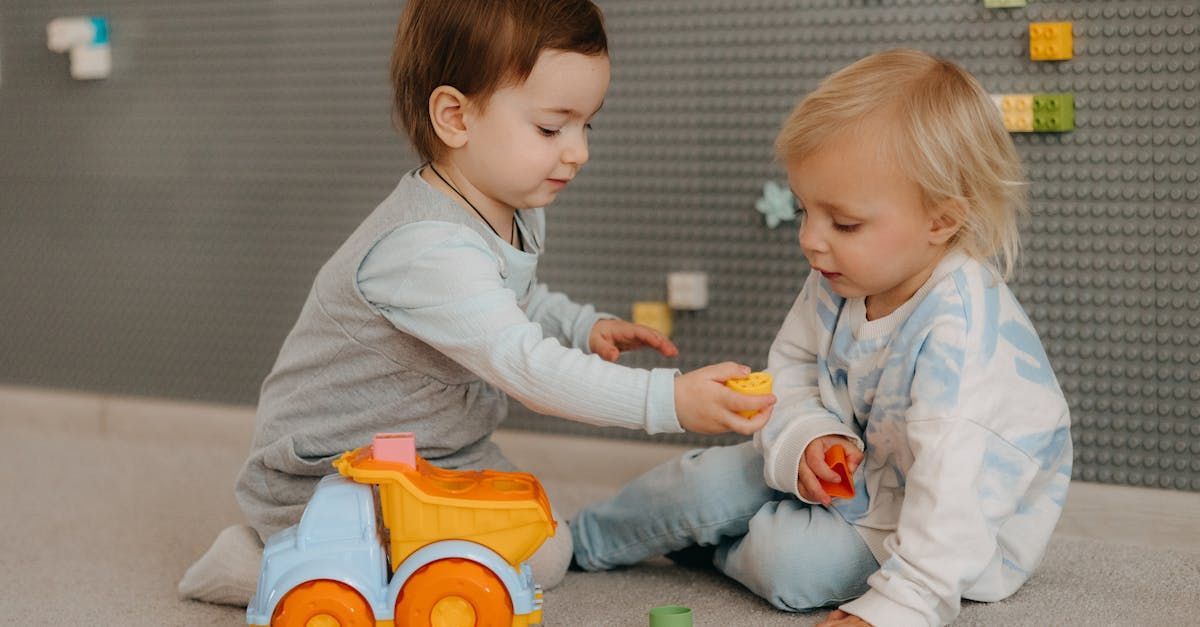
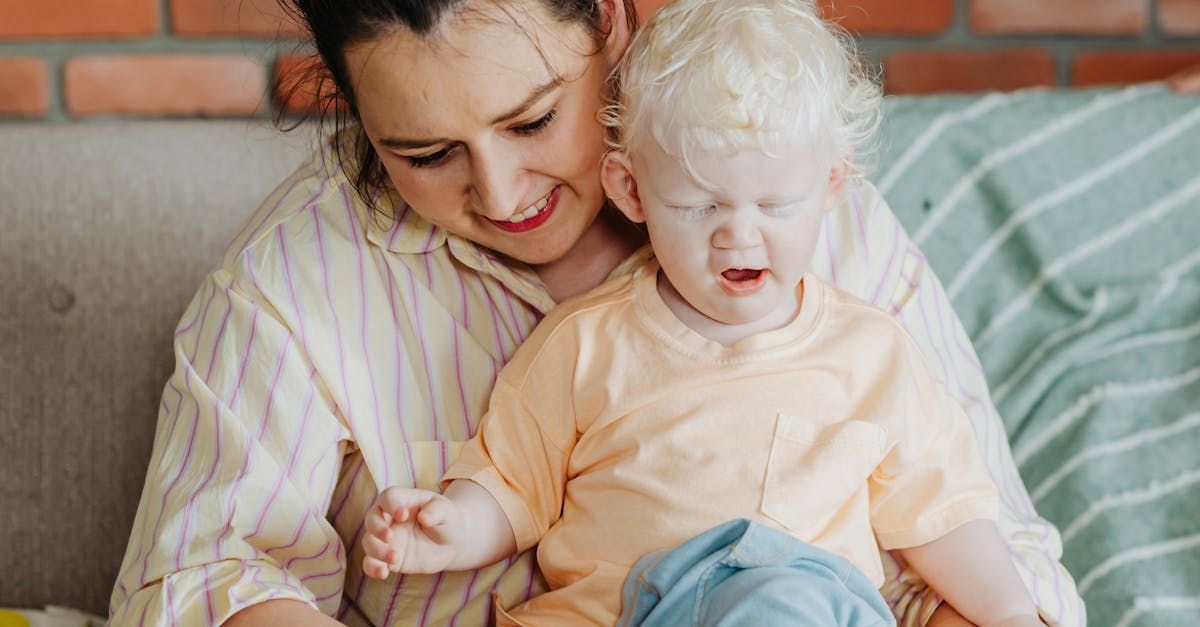
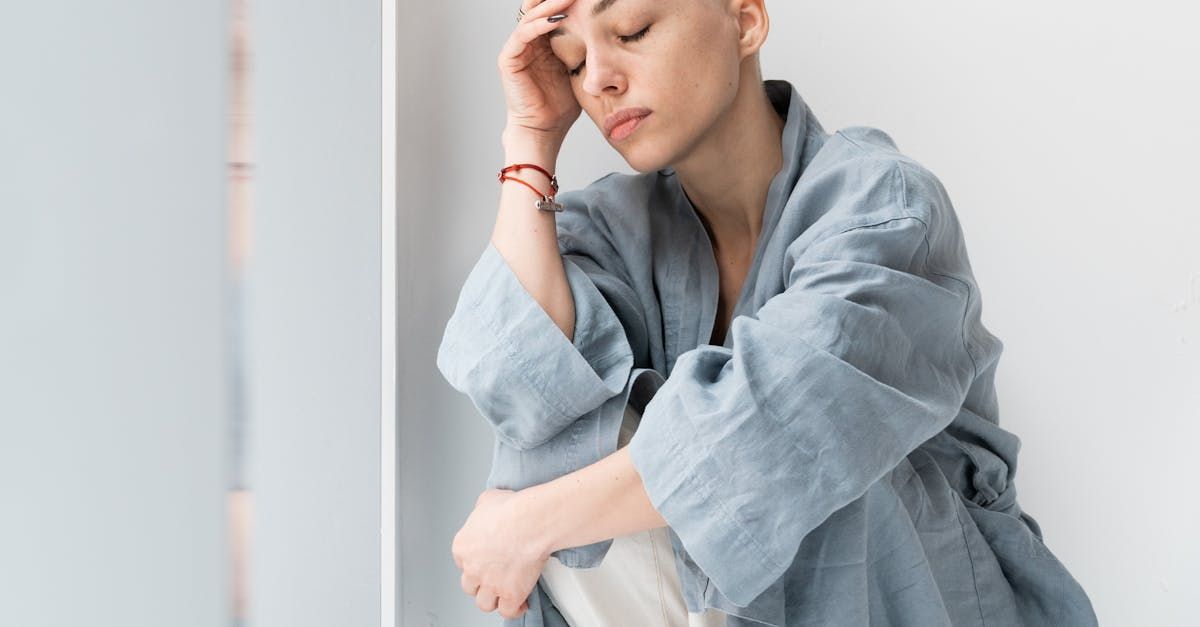
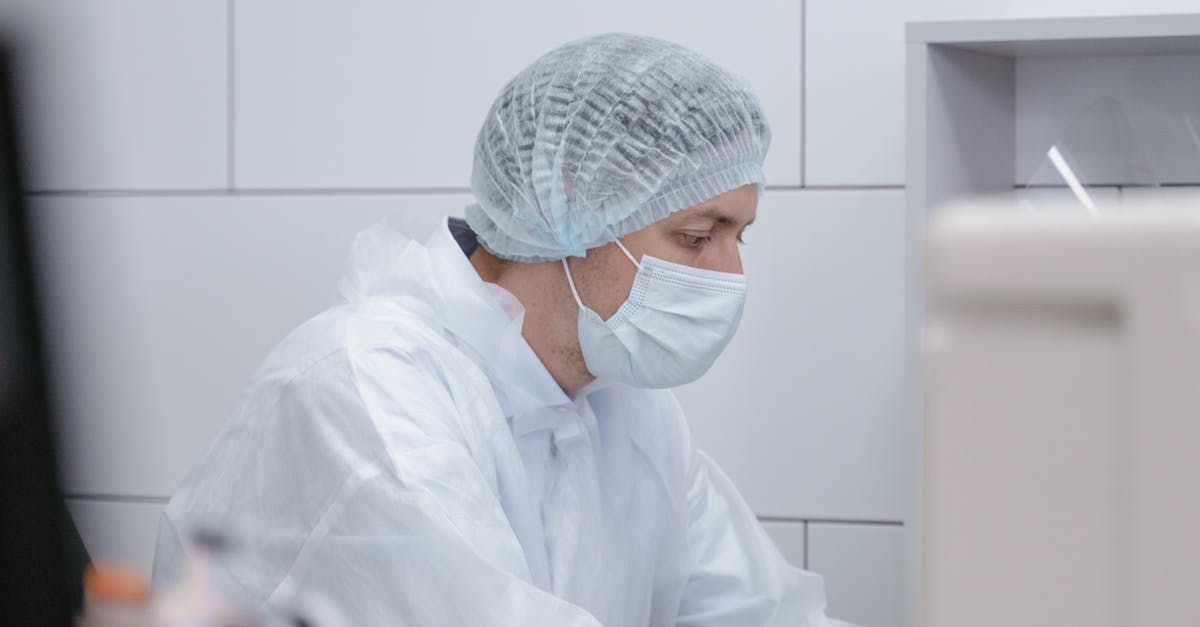
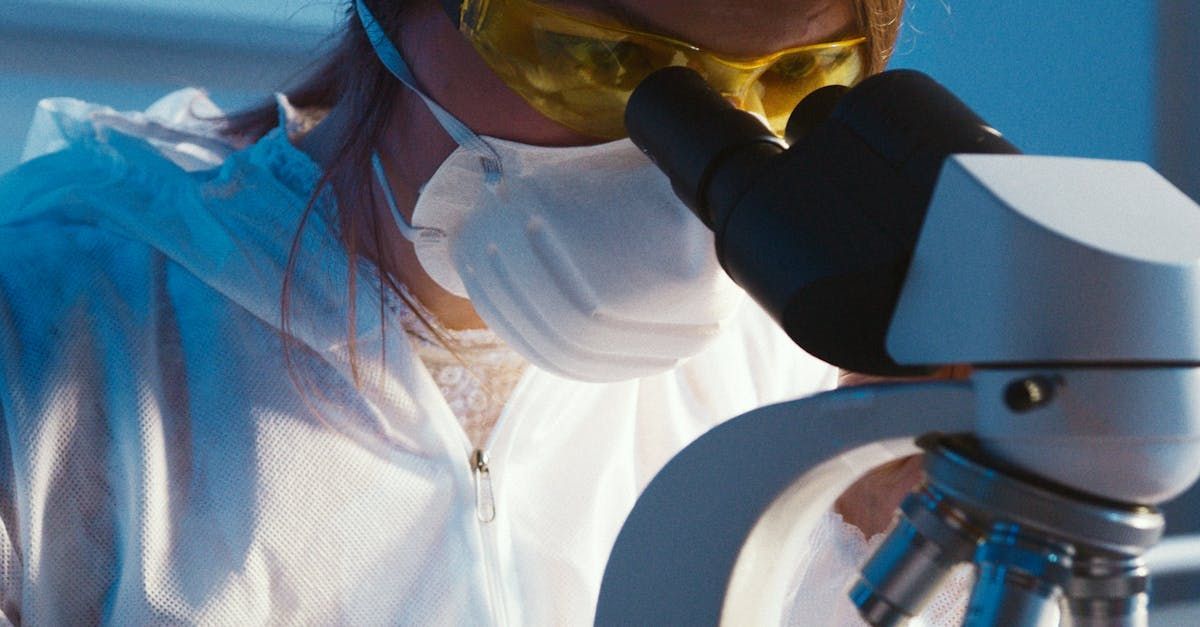
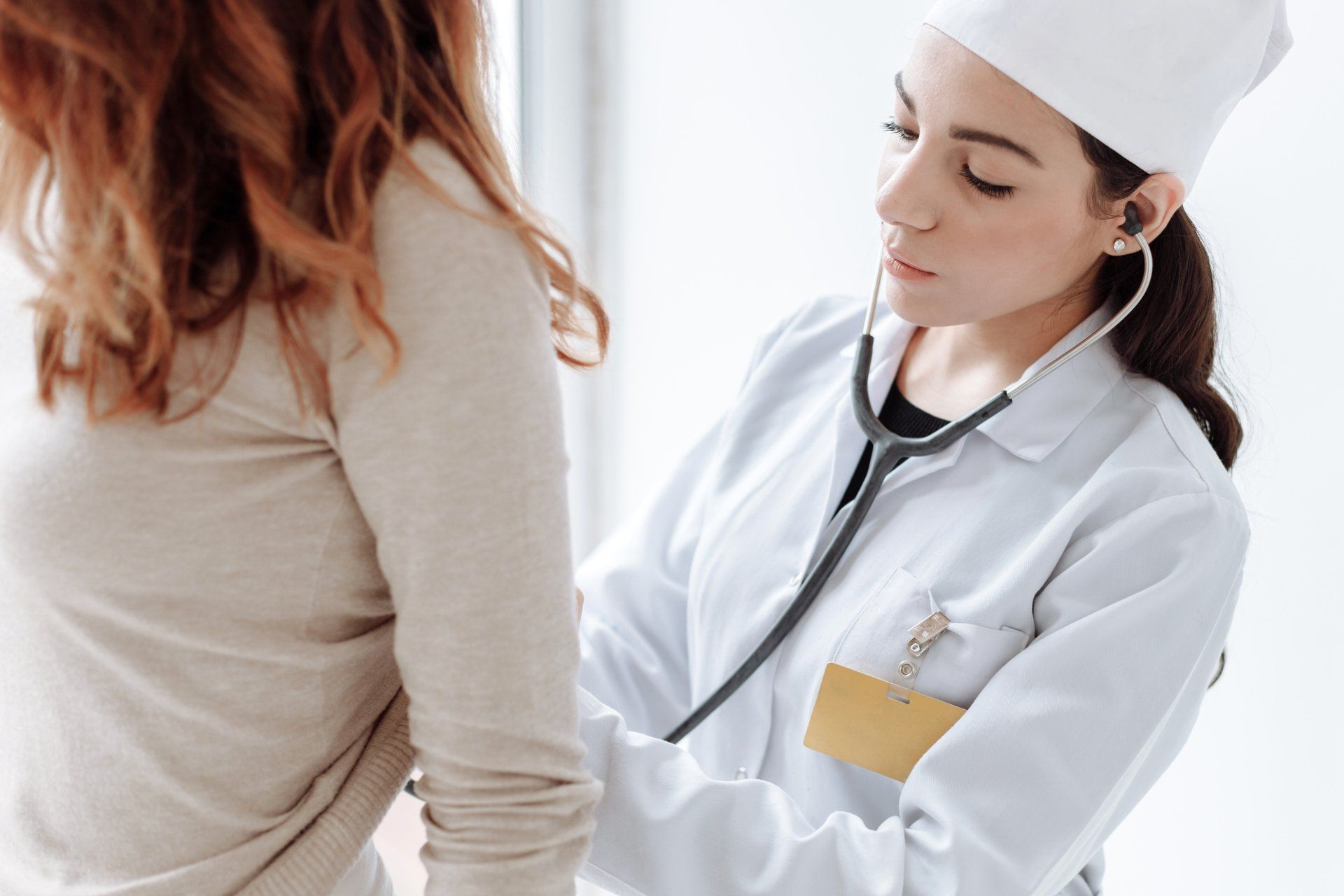
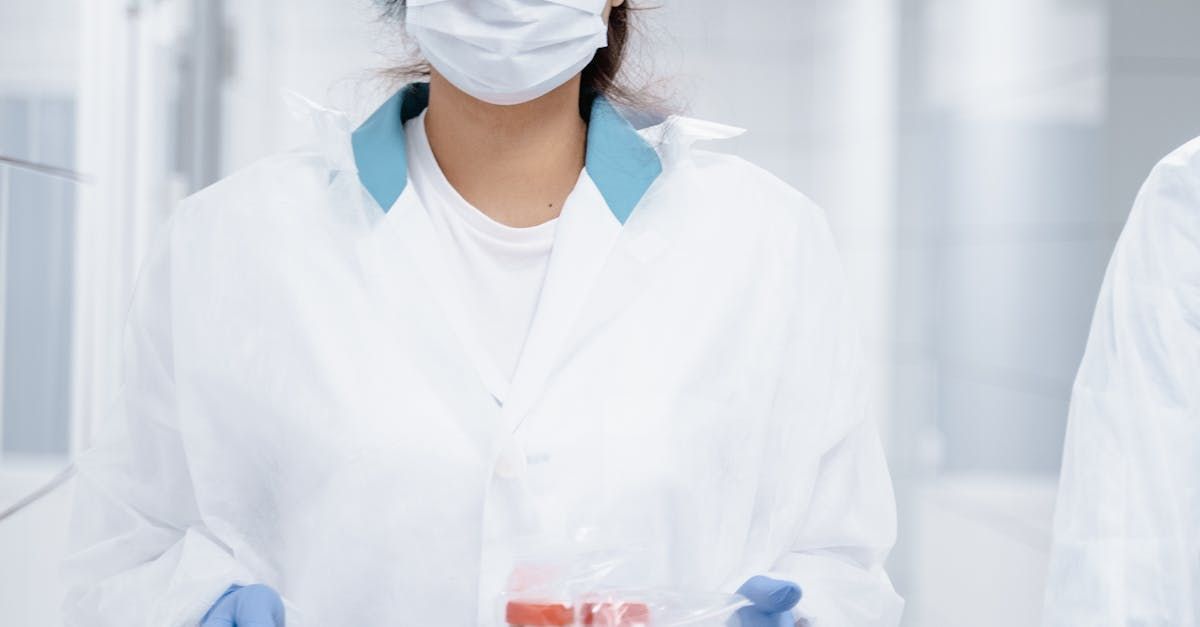