Leveraging Real-World Evidence in Clinical Trials
In the ever-evolving landscape of clinical research, the traditional boundaries of evidence collection and analysis are expanding. Real-world evidence (RWE) is emerging as a pivotal component in this transformation, offering insights that extend beyond the confines of controlled clinical trial environments. RWE encompasses data gathered from various sources outside of conventional randomized controlled trials (RCTs), such as electronic health records (EHRs), insurance claims, patient registries, wearable devices, and even social media.
The significance of RWE lies in its ability to provide a more comprehensive and realistic picture of how treatments perform in everyday settings. Unlike the highly controlled conditions of RCTs, real-world data reflects the diversity of patient populations, variations in clinical practice, and long-term outcomes. This broader perspective is invaluable for understanding the true impact of medical interventions on patient health and for identifying trends that might not be apparent in traditional trials.
The growing importance of RWE is underscored by its increasing acceptance by regulatory bodies like the U.S. Food and Drug Administration (FDA) and the European Medicines Agency (EMA). These organizations recognize the potential of RWE to enhance drug development, support regulatory decision-making, and improve post-market surveillance.
This blog delves into the role of real-world evidence in clinical trials, exploring how it supports drug approval processes and ongoing post-market monitoring. By examining case studies, regulatory perspectives, and the challenges and opportunities associated with RWE, we aim to shed light on its transformative potential in the realm of clinical research.
Understanding Real-World Evidence (RWE)
Real-world evidence (RWE) refers to the clinical evidence regarding the usage, benefits, and risks of a medical product derived from the analysis of real-world data (RWD). Unlike data collected in the highly controlled settings of randomized controlled trials (RCTs), RWD is gathered from routine clinical practice and other non-traditional sources. This data reflects the actual experiences of patients and healthcare providers, offering a more comprehensive view of how medical interventions perform in everyday life.
RWE is derived from a variety of sources, each contributing unique and valuable insights:
- Electronic Health Records (EHRs): EHRs provide detailed information on patient health histories, treatments, outcomes, and healthcare utilization. This data is instrumental in tracking long-term patient outcomes and identifying patterns in treatment effectiveness.
- Claims Data: Insurance claims data includes records of patient diagnoses, treatments, and healthcare services billed to insurance companies. This data helps in understanding healthcare utilization patterns and the economic impact of treatments.
- Patient Registries: Registries are organized systems that collect uniform data on patients with specific conditions or who have undergone particular treatments. These registries offer rich datasets for studying disease progression, treatment outcomes, and long-term safety.
- Wearable Devices: Wearables, such as fitness trackers and smartwatches, continuously collect data on various health metrics like physical activity, heart rate, and sleep patterns. This real-time data provides insights into how lifestyle factors influence health and treatment outcomes.
- Social Media: Platforms like Twitter, Facebook, and patient forums can be sources of patient-reported outcomes and experiences. Analyzing this data can reveal patient perspectives on treatments, adherence challenges, and quality of life issues.
Differences between RWE and Traditional Clinical Trial Data
The primary difference between RWE and traditional clinical trial data lies in their collection methods and contexts:
- Controlled Environment vs. Real-World Settings: RCTs are conducted in controlled environments with strict protocols to minimize variability and bias. In contrast, RWE is collected from real-world settings, reflecting the complexities and variations of everyday clinical practice.
- Patient Populations: RCTs often have stringent inclusion and exclusion criteria, leading to homogeneous patient populations. RWE encompasses a broader and more diverse patient population, including those with comorbidities and varying demographic characteristics.
- Data Collection Methods: RCT data is collected prospectively with predefined endpoints and standardized procedures. RWE is typically retrospective, utilizing data that was not collected for research purposes but rather for routine clinical care, insurance claims, or other real-world activities.
- Study Duration: RCTs are usually time-limited, focusing on short- to medium-term outcomes. RWE can provide insights into long-term and post-market effects of treatments, offering a more comprehensive view of their impact over time.
Understanding these differences is crucial for appreciating the complementary roles that RWE and traditional clinical trial data play in advancing medical research and improving patient care. By integrating RWE into the clinical trial framework, researchers can achieve a more holistic understanding of treatment efficacy and safety, ultimately leading to better-informed regulatory decisions and improved health outcomes.
The Role of RWE in Clinical Trials
Enhancing Clinical Trial Design
Real-world evidence (RWE) is playing an increasingly important role in enhancing clinical trial design. By integrating RWE, researchers can create more robust and comprehensive trials that better reflect the complexities of real-world medical practice. This integration allows for a more nuanced understanding of how treatments work across diverse patient populations and varied clinical settings.
Complementing Randomized Controlled Trials (RCTs)
While RCTs are the gold standard for determining the efficacy and safety of new treatments, they have limitations, such as high costs, lengthy timelines, and restrictive inclusion criteria. RWE can complement RCTs by providing additional context and insights that are often missing in controlled environments. For example, RWE can help identify patient subgroups that may benefit the most from a treatment or reveal long-term safety concerns that RCTs might not capture due to their limited duration.
Hybrid Trial Designs Combining RWE and RCT Data
One innovative approach to leveraging the strengths of both RWE and RCTs is through hybrid trial designs. These designs integrate real-world data with traditional clinical trial methodologies, creating a more flexible and comprehensive research framework. In a hybrid trial, RCT data provides a solid foundation of rigorous evidence, while RWE adds context and depth, enhancing the overall understanding of treatment effects. This approach can lead to more efficient trials, faster regulatory approvals, and more relevant findings for clinical practice.
Accelerating Patient Recruitment and Retention:
Patient recruitment and retention are significant challenges in clinical trials. RWE can address these issues by identifying potential participants through existing databases and registries, thereby speeding up the recruitment process. Additionally, by using data from real-world settings, researchers can design trials that are more patient-centric and less burdensome, improving retention rates. This patient-friendly approach can lead to higher quality data and more reliable trial outcomes.
Improving the Generalizability of Trial Results:
One of the key limitations of traditional RCTs is their limited generalizability due to strict inclusion and exclusion criteria. These criteria often result in study populations that do not fully represent the broader patient population. RWE, on the other hand, captures data from a wide range of patients in diverse clinical settings, enhancing the generalizability of trial results. By incorporating RWE, researchers can better understand how treatments perform across different demographics, comorbidities, and healthcare systems, making the findings more applicable to real-world clinical practice.
Supporting Drug Approval with RWE
Regulatory Acceptance of RWE by FDA and EMA
The use of real-world evidence (RWE) in the regulatory landscape has gained significant traction, particularly with agencies like the U.S. Food and Drug Administration (FDA) and the European Medicines Agency (EMA). Both regulatory bodies have recognized the potential of RWE to complement traditional clinical trial data and support drug approval processes. The FDA’s 21st Century Cures Act and the EMA’s Adaptive Pathways initiative exemplify the growing acceptance of RWE. These frameworks encourage the integration of RWE to enhance decision-making, particularly in areas where traditional data might be limited, such as rare diseases or post-market surveillance.
Case Studies of Successful Drug Approvals Using RWE
Several case studies highlight the successful use of RWE in drug approvals, demonstrating its value in providing comprehensive evidence of a treatment's effectiveness and safety:
- Palbociclib (Ibrance): The FDA approved Palbociclib for breast cancer treatment based on a combination of RCT data and RWE. The additional real-world data helped illustrate the drug's performance in broader, more diverse populations, supporting its safety and efficacy beyond the controlled trial environment.
- Blincyto (blinatumomab): Blincyto, used for treating a specific type of leukemia, received accelerated approval from the FDA. RWE played a crucial role by providing post-market data that demonstrated long-term benefits and supported the initial findings from RCTs.
- Real-world evidence (RWE) is playing an increasingly vital role in clinical trials by providing insights derived from real-world data, such as electronic health records, patient registries, and other health-related data collected outside of traditional clinical settings. Leveraging RWE in clinical trials can enhance the understanding of treatment effectiveness and safety in diverse, real-world populations, thereby supporting more informed decision-making in drug development and regulatory approval processes. Notable Labs, for instance, utilizes RWE to refine patient selection and improve trial outcomes through their Predictive Precision Medicine Platform.
These case studies underscore how RWE can provide valuable insights that complement traditional clinical trial data, enhancing the robustness of the evidence submitted for regulatory approval.
Key Considerations for Using RWE in Regulatory Submissions
While RWE offers significant advantages, its use in regulatory submissions requires careful consideration of several key factors to ensure the data meets the rigorous standards expected by regulatory bodies.
Data Quality and Integrity:
High-quality, reliable data is paramount for regulatory acceptance of RWE. Ensuring data quality involves:
- Data Sources: Selecting credible and comprehensive data sources, such as well-maintained electronic health records (EHRs) or validated patient registries.
- Data Standardization: Implementing standardized data collection methods to minimize variability and ensure consistency across different data sources.
- Data Validation: Conducting thorough data validation processes to verify the accuracy and completeness of the real-world data used in analyses.
Methodological Rigor:
The methodological approach to analyzing RWE is critical for its acceptance in regulatory submissions. Key aspects include:
- Study Design: Employing robust study designs that account for potential biases and confounding factors inherent in real-world data. This may involve techniques such as propensity score matching or instrumental variable analysis.
- Statistical Analysis: Utilizing appropriate statistical methods to analyze RWE, ensuring that the results are scientifically sound and reliable.
- Transparency and Reproducibility: Providing detailed documentation of the study design, data sources, and analytical methods to allow for independent verification and reproducibility of the findings.
By addressing these considerations, researchers can enhance the credibility and regulatory acceptance of RWE, facilitating its use in drug approval processes.
Post-Market Surveillance and RWE
Importance of Post-Market Surveillance
Post-market surveillance is a critical component of the drug development lifecycle. While pre-market clinical trials provide initial evidence of a drug’s safety and efficacy, these trials are often limited in duration and scope. Post-market surveillance ensures that the safety and effectiveness of a drug are continuously monitored after it has been approved and is widely used. This ongoing surveillance helps identify any adverse events or long-term effects that may not have been apparent during the pre-approval phase, thus safeguarding public health and ensuring that patients benefit from the best possible treatments.
Using RWE for Ongoing Monitoring of Drug Safety and Efficacy
Real-world evidence (RWE) is invaluable for post-market surveillance. By leveraging data from electronic health records (EHRs), insurance claims, patient registries, wearables, and other real-world sources, researchers and regulatory bodies can monitor the performance of a drug in a larger and more diverse patient population. This ongoing monitoring allows for the detection of rare adverse events, assessment of long-term safety and efficacy, and identification of any emerging safety signals. RWE provides a more comprehensive understanding of a drug’s impact in real-world clinical practice, enabling timely interventions if any issues are detected.
Examples of Post-Market Studies Utilizing RWE
Several post-market studies have effectively utilized RWE to monitor drug safety and efficacy:
- Rivaroxaban (Xarelto): Post-market surveillance using RWE identified an increased risk of major bleeding events associated with rivaroxaban, an anticoagulant medication. The findings from real-world data prompted further investigation and led to updated safety recommendations and label changes.
- Human Papillomavirus (HPV) Vaccines: RWE has been used to monitor the long-term safety and effectiveness of HPV vaccines. Large-scale studies using data from national health registries have confirmed the vaccines' role in reducing the incidence of HPV-related cancers and precancerous lesions, providing robust evidence of their public health impact.
These examples demonstrate how RWE can provide critical insights into the long-term safety and effectiveness of medical treatments, supporting proactive risk management and enhancing patient care.
Benefits of RWE in Identifying Long-Term Effects and Rare Adverse Events
One of the key strengths of RWE is its ability to identify long-term effects and rare adverse events that may not be captured in pre-market clinical trials:
- Long-Term Effects: RWE enables the monitoring of patients over extended periods, providing insights into the long-term benefits and risks of treatments. This long-term data is essential for understanding the true impact of a drug on patient health and quality of life.
- Rare Adverse Events: Due to the larger and more diverse patient populations included in RWE studies, rare adverse events that were not detected in smaller, pre-market trials can be identified. This comprehensive safety monitoring is crucial for ensuring that all potential risks associated with a drug are understood and managed.
Challenges and Limitations
Data Privacy and Security Concerns
One of the primary challenges in utilizing real-world evidence (RWE) is ensuring the privacy and security of patient data. Real-world data (RWD) is often derived from sources like electronic health records (EHRs), insurance claims, and wearable devices, which contain sensitive personal health information. Protecting this data from unauthorized access and breaches is paramount. Regulatory frameworks such as the Health Insurance Portability and Accountability Act (HIPAA) in the United States and the General Data Protection Regulation (GDPR) in Europe set stringent requirements for data privacy and security, but compliance can be complex and resource-intensive.
Variability and Heterogeneity of Real-World Data
Real-world data is inherently diverse and heterogeneous, reflecting the varied practices and patient populations across different healthcare settings. This variability can complicate data analysis and interpretation. Differences in data quality, formats, and completeness across sources can lead to inconsistencies and potential inaccuracies in RWE studies. Standardizing data collection and processing methods is essential to ensure that RWE is reliable and comparable across studies.
Potential Biases in RWE Studies
RWE studies can be susceptible to various biases that may affect the validity of their findings. Common biases include:
- Selection Bias: Patients included in RWE studies may not be representative of the general population, particularly if the data sources have specific inclusion criteria or if certain groups are underrepresented.
- Confounding: Real-world settings often involve multiple factors that can influence outcomes, making it challenging to isolate the effect of the treatment being studied.
- Information Bias: Inaccuracies or inconsistencies in the recording of data can lead to misclassification and erroneous conclusions.
Strategies to Address and Mitigate These Challenges
To maximize the potential of RWE while addressing its challenges, several strategies can be employed:
- Enhanced Data Privacy and Security Measures: Implementing robust encryption, access controls, and anonymization techniques can help protect patient data. Adopting best practices for data governance and compliance with relevant regulatory requirements is also crucial.
- Standardization and Data Quality Improvement: Developing and adhering to standardized protocols for data collection, processing, and analysis can mitigate the variability and heterogeneity of RWD. Efforts such as the adoption of common data models and the establishment of data quality benchmarks can enhance the consistency and reliability of RWE.
- Bias Mitigation Techniques: Employing advanced statistical methods and study designs can help control for biases in RWE studies. Techniques such as propensity score matching, instrumental variable analysis, and sensitivity analyses can reduce the impact of confounding factors. Ensuring diverse and representative patient samples can also address selection bias.
- Transparency and Documentation: Providing detailed documentation of data sources, study methodologies, and analytical approaches enhances the transparency and reproducibility of RWE studies. This transparency allows for independent validation and increases confidence in the findings.
By addressing these challenges through rigorous methodologies and best practices, the reliability and credibility of RWE can be significantly enhanced. This, in turn, ensures that RWE continues to play a valuable role in informing clinical and regulatory decision-making, ultimately benefiting patient care and advancing medical research.
Future Directions and Opportunities
Technological Advancements Enhancing RWE Collection and Analysis
Technological advancements are rapidly transforming the collection and analysis of real-world evidence (RWE). Innovations such as artificial intelligence (AI) and machine learning (ML) are enabling more sophisticated data analytics, allowing researchers to extract meaningful insights from vast and complex datasets. Natural language processing (NLP) techniques are improving the ability to analyze unstructured data from clinical notes, social media, and other sources. Additionally, the proliferation of wearable devices and mobile health applications is providing continuous, real-time health data, offering a richer and more dynamic understanding of patient health and treatment outcomes.
Integration of RWE into Precision Medicine Initiatives
The integration of RWE into precision medicine initiatives represents a significant opportunity for personalized healthcare. By leveraging real-world data, researchers can better understand how different treatments work for specific patient subgroups, considering genetic, environmental, and lifestyle factors. This personalized approach can lead to more effective and tailored therapies, reducing trial-and-error in treatment selection and improving patient outcomes. RWE can also help identify biomarkers and other predictors of treatment response, further advancing the field of precision medicine.
Collaboration Between Stakeholders: Researchers, Regulators, and Healthcare Providers
The successful integration and utilization of RWE require close collaboration between various stakeholders, including researchers, regulators, and healthcare providers. Collaborative efforts can ensure that RWE is collected, analyzed, and used in ways that maximize its value for clinical and regulatory decision-making. Establishing common data standards, sharing best practices, and creating collaborative data networks can enhance the quality and consistency of RWE. Regulatory bodies can provide guidance and frameworks that facilitate the use of RWE in drug development and approval processes, while healthcare providers can contribute valuable real-world data and clinical insights.
Potential for RWE to Transform Clinical Trial Paradigms
The potential for RWE to transform clinical trial paradigms is immense. By integrating RWE into traditional trial designs, researchers can develop more flexible and adaptive trial methodologies that better reflect real-world conditions. Pragmatic clinical trials, which incorporate real-world settings and patient populations, can provide more generalizable and applicable results. Additionally, RWE can support decentralized clinical trials, reducing the burden on participants and increasing access to diverse populations. This transformation can lead to more efficient and patient-centered research, accelerating the development of new therapies and improving healthcare outcomes.
Recap of the key points discussed
Throughout this blog, we have explored the transformative potential of real-world evidence (RWE) in clinical trials and drug development. We began by defining RWE and identifying its diverse sources, such as electronic health records (EHRs), claims data, patient registries, wearables, and social media. We highlighted the differences between RWE and traditional clinical trial data, emphasizing how RWE provides a more comprehensive view of treatment effects in real-world settings.
We then examined the role of RWE in enhancing clinical trial design, complementing randomized controlled trials (RCTs), and enabling hybrid trial designs. We discussed how RWE can accelerate patient recruitment and retention and improve the generalizability of trial results.
Next, we delved into the regulatory acceptance of RWE by agencies like the FDA and EMA, and presented case studies of successful drug approvals using RWE. We outlined key considerations for using RWE in regulatory submissions, including data quality, integrity, and methodological rigor.
In the context of post-market surveillance, we highlighted the importance of ongoing monitoring of drug safety and efficacy using RWE. We provided examples of post-market studies that have utilized RWE to identify long-term effects and rare adverse events, underscoring the benefits of this approach.
We also addressed the challenges and limitations associated with RWE, such as data privacy and security concerns, variability and heterogeneity of real-world data, and potential biases in RWE studies. Strategies to mitigate these challenges were discussed, emphasizing the importance of data quality, standardization, and transparency.
Finally, we explored future directions and opportunities for RWE, including technological advancements, integration into precision medicine initiatives, collaboration between stakeholders, and the potential to transform clinical trial paradigms.
The significance of RWE in clinical trials and drug development is rapidly growing. As healthcare systems increasingly generate and leverage real-world data, the ability to capture and analyze this information becomes crucial for advancing medical research and improving patient outcomes. RWE provides a more holistic view of how treatments perform in diverse, real-world settings, complementing the findings from traditional clinical trials and enhancing our understanding of treatment efficacy and safety.
Regulatory bodies are recognizing the value of RWE, and its acceptance in regulatory submissions is expanding. This shift is facilitating more flexible and efficient drug development processes, ultimately accelerating the availability of new therapies for patients. Additionally, the use of RWE in post-market surveillance is ensuring that the safety and effectiveness of treatments are continuously monitored, contributing to better patient care and public health.
The future impact of RWE in healthcare and pharmaceutical research is promising. Technological advancements, such as AI and machine learning, will continue to enhance the collection and analysis of real-world data, providing deeper insights into patient health and treatment outcomes. The integration of RWE into precision medicine initiatives will drive the development of more personalized therapies, tailored to the unique characteristics of individual patients.
Collaboration between researchers, regulators, and healthcare providers will be essential for maximizing the value of RWE and ensuring its successful application in clinical and regulatory contexts. By working together, these stakeholders can establish common data standards, share best practices, and create collaborative data networks that enhance the quality and consistency of RWE.
As RWE continues to evolve, it has the potential to fundamentally transform clinical trial paradigms, making them more patient-centered, efficient, and reflective of real-world conditions. This transformation will lead to more effective and personalized treatments, improved patient outcomes, and a more responsive healthcare system.
In conclusion, the integration of RWE into clinical research and drug development represents a significant opportunity for advancing healthcare. By embracing the potential of real-world data and addressing its challenges with methodological rigor and transparency, we can create a future where evidence-based medicine is more personalized, effective, and reflective of the complexities of real-world clinical practice. This evolution will ultimately benefit patients, healthcare providers, and the broader healthcare ecosystem, contributing to a healthier and more informed society.
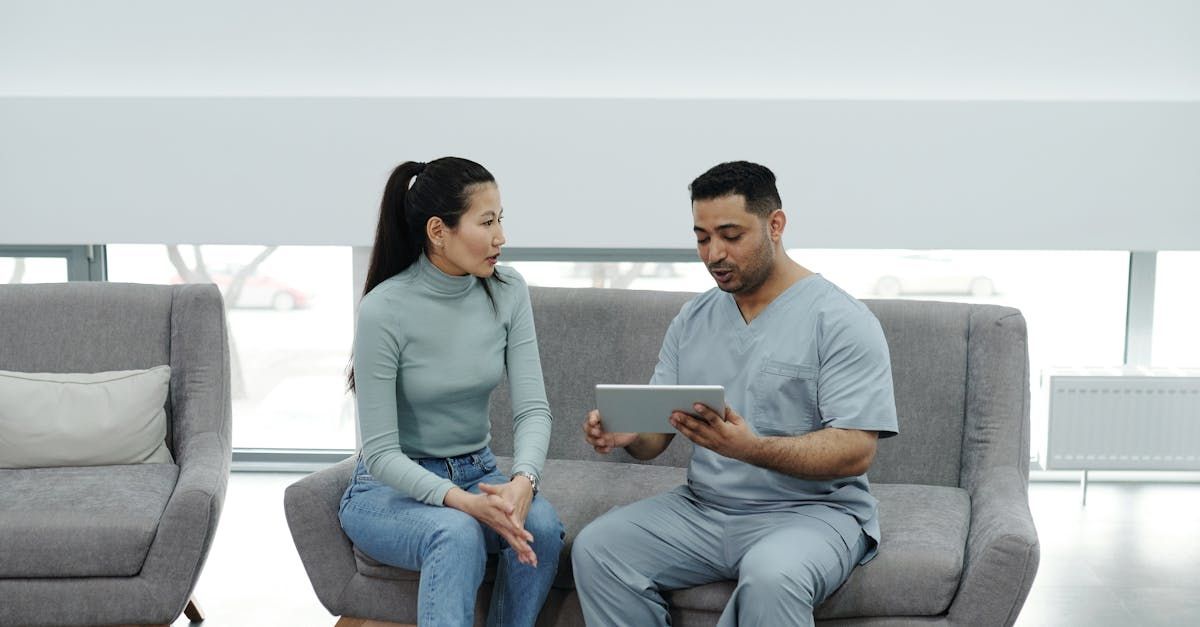
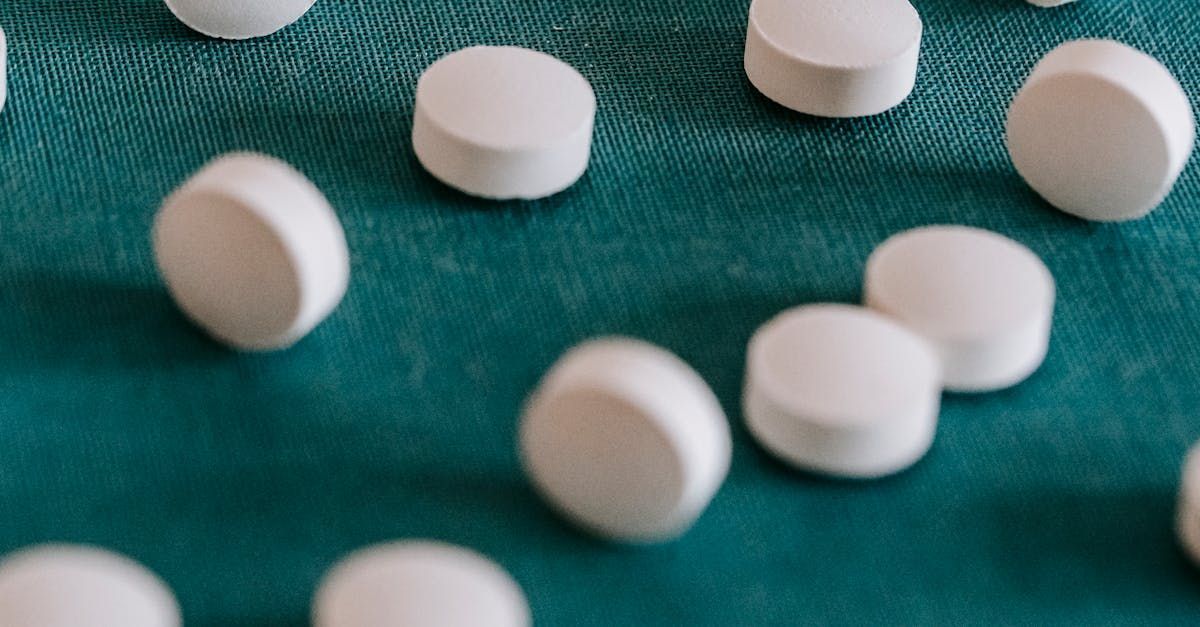
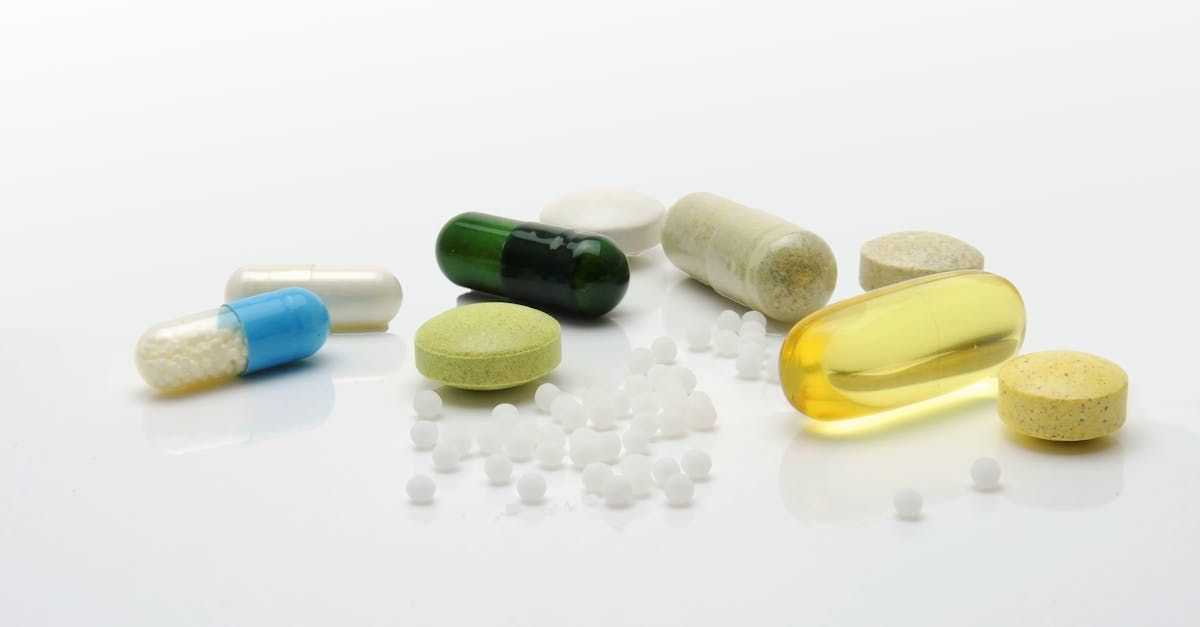
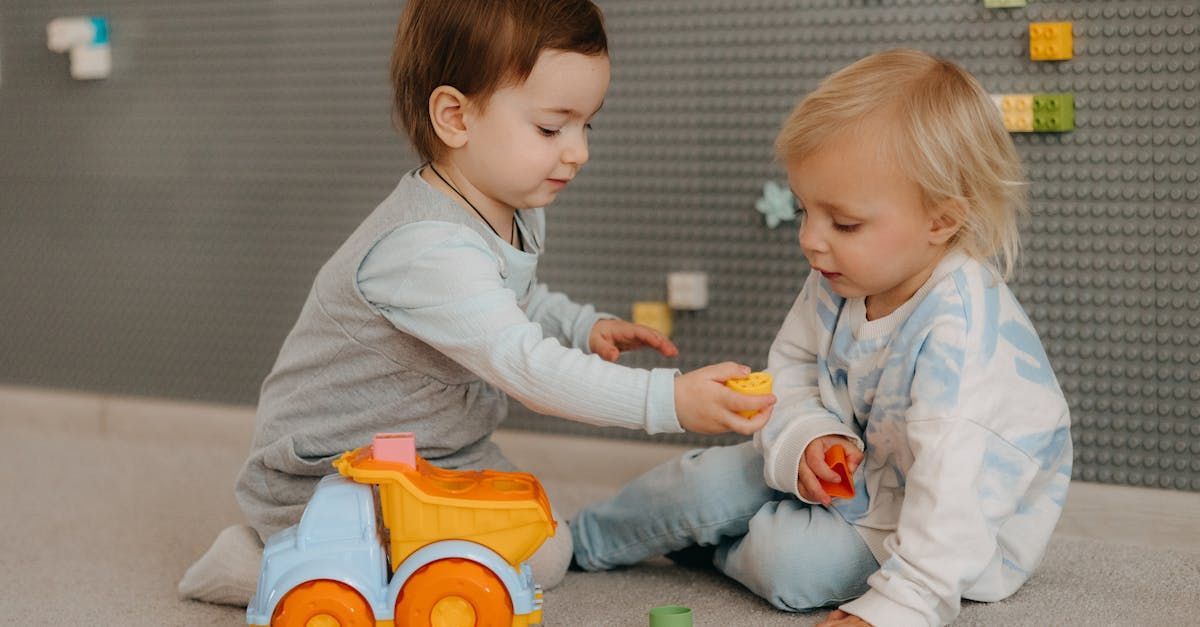
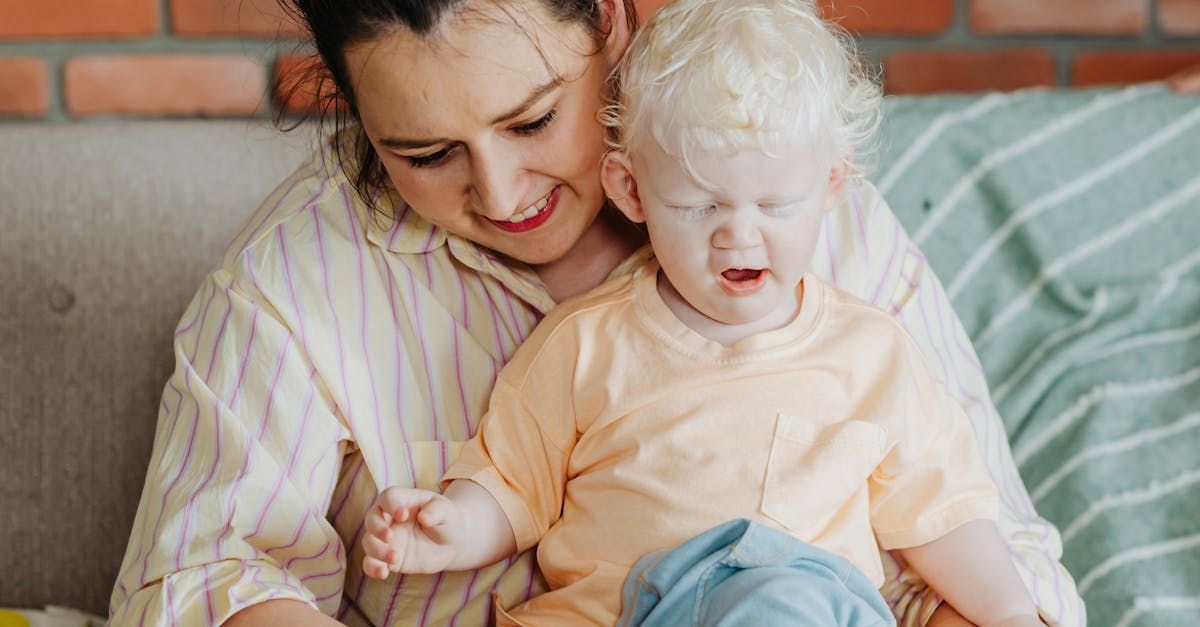
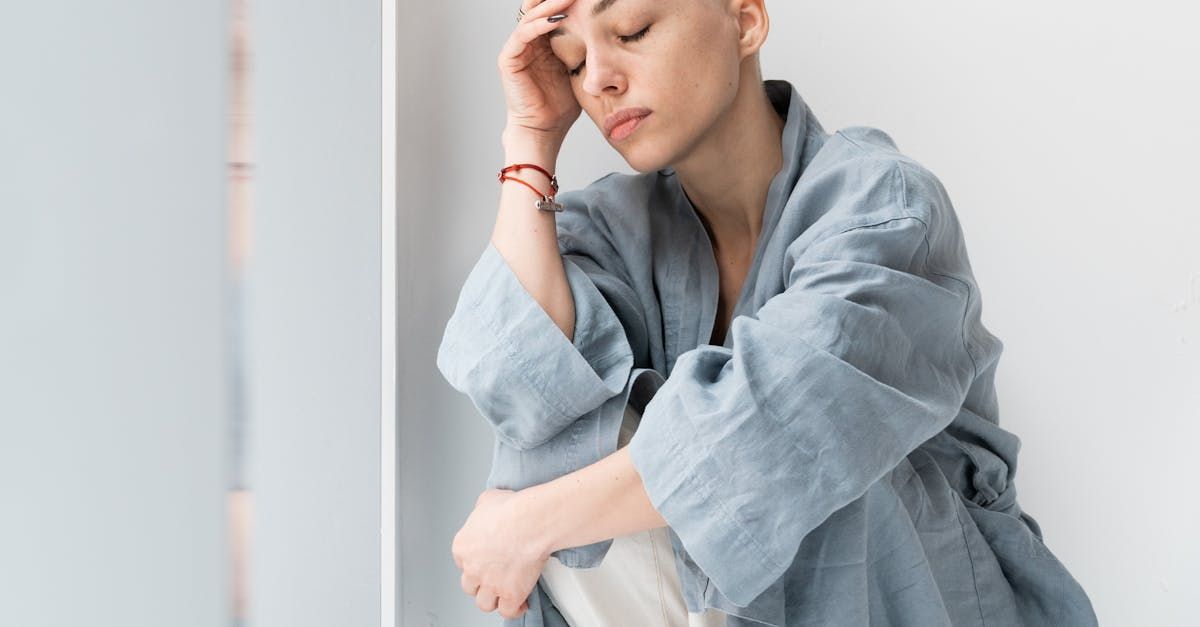
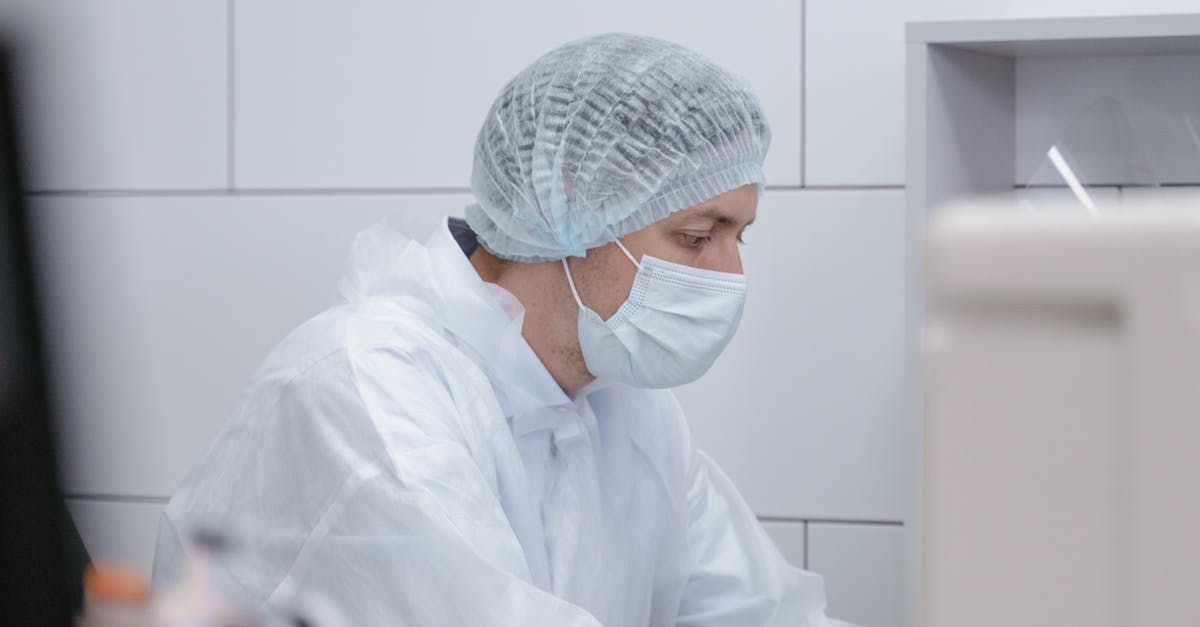
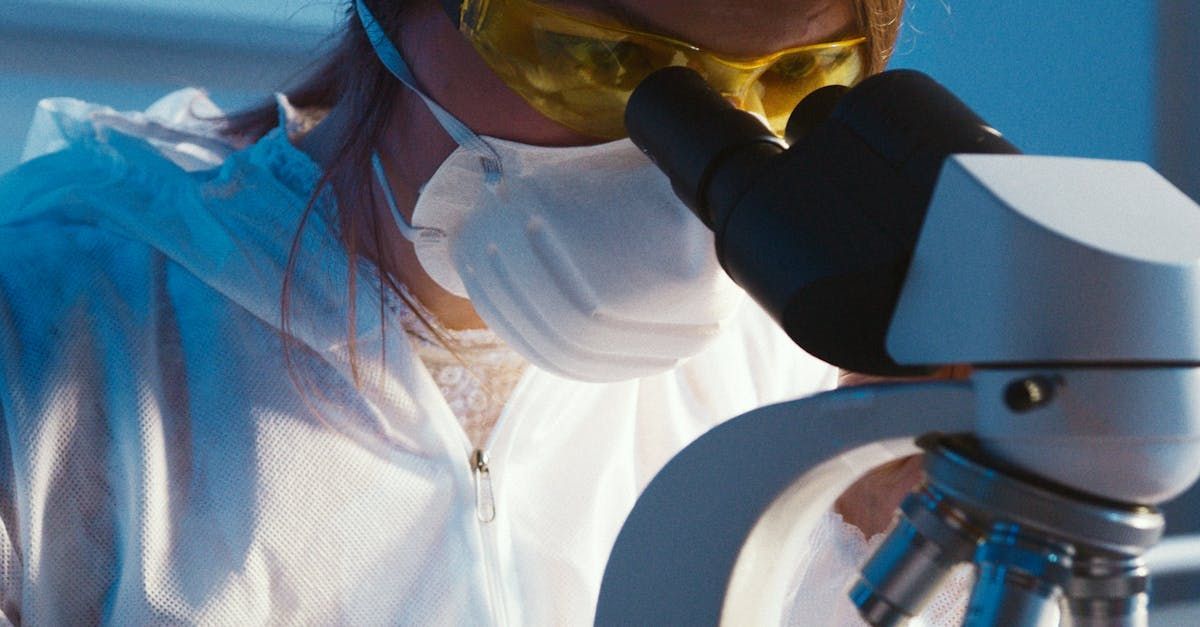
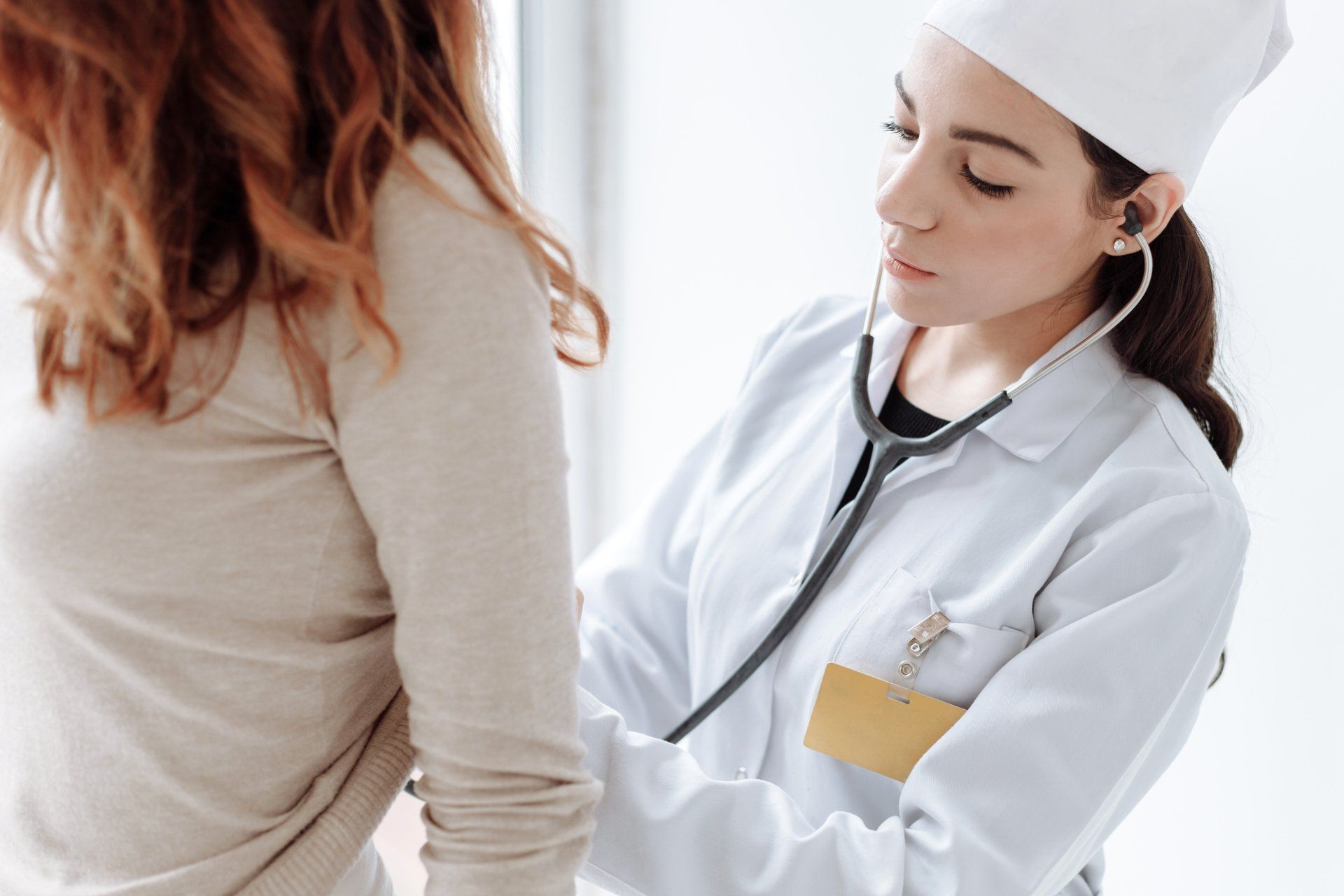
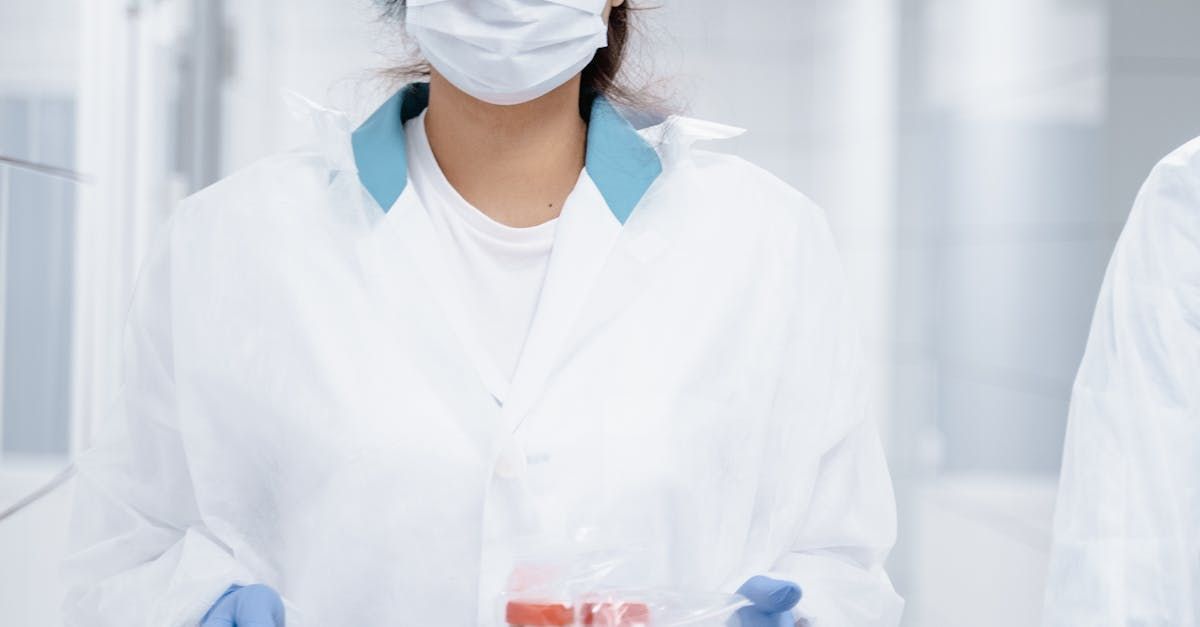