The Role of Real-World Data in Precision Medicine
Precision medicine represents a transformative approach in healthcare, where medical treatment is tailored to the individual characteristics of each patient. This paradigm shift focuses on the unique genetic makeup, lifestyle, and environmental factors of each person, allowing for more precise and effective interventions. Unlike traditional one-size-fits-all medicine, precision medicine aims to maximize therapeutic benefits while minimizing potential risks, by considering the variability in genes, environment, and lifestyle for each individual.
The goals of precision medicine are ambitious and far-reaching. They include the development of more effective therapies, the identification of individuals at risk for certain diseases before symptoms appear, and the tailoring of preventive measures and treatments to the individual. This approach is particularly significant in the management of complex diseases such as cancer, cardiovascular diseases, and rare genetic disorders. By targeting therapies based on a deeper understanding of the biological mechanisms at play in each patient, precision medicine holds the promise of improving health outcomes and quality of life.
A crucial element in the implementation of precision medicine is the use of data. Specifically, the integration of real-world data (RWD) alongside traditional clinical trial data has emerged as a key component in this personalized approach to healthcare. RWD provides insights from a wide range of sources outside the controlled environment of clinical trials, offering a more comprehensive view of how treatments work in everyday settings. In this blog, we will explore the role of RWD in precision medicine, how it complements clinical trial data, and the challenges and opportunities it presents.
Understanding Real-World Data (RWD)
Real-world data encompasses information collected from various sources that reflect the everyday experiences of patients. This data can come from electronic health records (EHRs), insurance claims, patient registries, wearable devices, and even social media platforms. Unlike clinical trial data, which is gathered in controlled settings with strict protocols, RWD captures the diverse and often messy reality of medical practice and patient behavior.
The types of RWD are varied. Electronic health records provide detailed information on patient demographics, diagnoses, treatments, and outcomes. Insurance claims data can offer insights into healthcare utilization and costs. Patient registries often focus on specific diseases or conditions, collecting data on patient characteristics, treatments, and outcomes over time. Additionally, patient-reported outcomes collected through surveys or wearable devices can provide information on quality of life, adherence to treatments, and other aspects not typically captured in clinical settings.
The differences between RWD and clinical trial data are significant. Clinical trials are conducted under highly controlled conditions to ensure that the results are attributable to the interventions being tested. Participants are often carefully selected to meet specific criteria, and the environment is meticulously managed to minimize confounding factors. As a result, clinical trial data can be limited in terms of generalizability. In contrast, RWD is collected in the real world, where patients may have multiple comorbidities, take various medications, and face different socio-economic and environmental factors. This makes RWD more representative of the broader patient population but also more complex to analyze and interpret.
The Complementary Role of Real-World Data in Precision Medicine
The use of RWD in precision medicine addresses some of the key limitations of clinical trial data. One of the primary challenges with clinical trial data is its generalizability. Clinical trials often involve a relatively small and homogeneous group of participants, which can limit the applicability of the findings to the broader patient population. For example, clinical trials may underrepresent certain demographic groups such as the elderly, minorities, or those with multiple health conditions. This limitation can make it difficult to apply trial findings to these underrepresented groups.
RWD helps to overcome these limitations by providing data from a much larger and more diverse population. This broader representation allows for a better understanding of how treatments work across different demographic and socio-economic groups, enhancing the ability to tailor treatments to individual patients. Moreover, RWD can capture long-term outcomes and rare side effects that may not be apparent during the relatively short duration of clinical trials. This information is critical for understanding the real-world effectiveness and safety of treatments.
In precision medicine, the integration of RWD with clinical trial data is essential for developing personalized treatment protocols. By combining data from both sources, healthcare providers can gain a more comprehensive view of a patient's health status and potential responses to treatment. For example, clinical trial data may provide information on the efficacy of a drug in a controlled setting, while RWD can offer insights into its effectiveness and safety in everyday use. This combination of data sources allows for a more nuanced approach to treatment, taking into account the complexities of real-world patients.
Advanced analytics play a crucial role in integrating and analyzing RWD. Techniques such as big data analytics, machine learning, and artificial intelligence are increasingly used to sift through vast amounts of RWD, identifying patterns and generating insights that would be difficult to discern through traditional methods. These technologies can help to identify patient subgroups that are likely to benefit from specific treatments, predict patient outcomes, and even suggest new therapeutic targets. By leveraging these tools, researchers and clinicians can make more informed decisions, improving the precision and effectiveness of medical interventions.
Case Studies: Integration of Real-World Data in Clinical Trials and Outcomes
The integration of RWD in clinical trials and outcomes has already shown significant benefits in various fields of medicine. Here, we explore three case studies that highlight the impact of RWD in oncology, cardiovascular diseases, and rare diseases.
Case Study 1: Oncology
In oncology, RWD has been instrumental in identifying patient subgroups that can benefit from targeted therapies. For example, in the treatment of lung cancer, RWD has been used to identify patients with specific genetic mutations, such as EGFR or ALK mutations, who are more likely to respond to targeted therapies. These therapies, which are designed to attack cancer cells with specific genetic abnormalities, have been shown to be more effective and have fewer side effects compared to traditional chemotherapy.
A notable success story is the use of RWD to match patients with the drug pembrolizumab, an immune checkpoint inhibitor. Clinical trial data initially demonstrated its effectiveness in patients with advanced melanoma, but RWD later revealed its broader potential. By analyzing RWD, researchers identified additional cancer types and patient subgroups that could benefit from pembrolizumab, leading to expanded FDA approvals and more personalized treatment options for patients.
Case Study 2: Cardiovascular Diseases
In the field of cardiovascular diseases, RWD has been used to monitor the long-term outcomes of interventions and treatments. For example, the use of RWD has been crucial in understanding the effectiveness and safety of statins, a class of drugs used to lower cholesterol levels and reduce the risk of heart disease. While clinical trials provided initial evidence of their benefits, RWD has been invaluable in monitoring their long-term effects in a more diverse population, including patients with multiple comorbidities.
An example of how RWD has influenced the management of heart failure patients is the use of implantable cardioverter-defibrillators (ICDs). Clinical trials demonstrated the efficacy of ICDs in preventing sudden cardiac death in patients with severe heart failure. However, RWD has provided insights into the real-world usage patterns, adherence to device therapy, and long-term outcomes, helping to refine patient selection criteria and improve overall patient care.
Case Study 3: Rare Diseases
Rare diseases often lack comprehensive clinical trial data due to the small number of affected patients. In these cases, RWD can be particularly valuable. For instance, in the treatment of rare genetic disorders such as cystic fibrosis, RWD has been used to identify off-label uses of existing drugs that may benefit patients. By analyzing data from patient registries and EHRs, researchers have been able to identify patterns of drug use and outcomes, leading to new treatment options and improved patient care.
A specific example involves the use of the drug ivacaftor for cystic fibrosis patients with rare CFTR mutations. Initially approved for a limited set of mutations based on clinical trial data, subsequent analysis of RWD revealed its effectiveness in a broader range of mutations. This finding led to expanded use and approvals, significantly improving the quality of life for many patients with cystic fibrosis.
Notable Labs: Leveraging Real-World Data for Precision Medicine
Notable Labs is at the forefront of integrating real-world data (RWD) to revolutionize precision medicine. Precision medicine tailors medical treatment to the unique characteristics of each patient, considering their genetic makeup, lifestyle, and environmental factors. This approach aims to deliver more precise and effective interventions, moving away from the traditional one-size-fits-all model.
The goals of precision medicine are ambitious, including developing more effective therapies, identifying individuals at risk for certain diseases, and tailoring preventive measures and treatments. This is particularly important for managing complex diseases like cancer, cardiovascular diseases, and rare genetic disorders. By targeting therapies based on a deeper understanding of each patient's biological mechanisms, precision medicine can significantly improve health outcomes and quality of life.
Real-world data is crucial in achieving these goals. Unlike clinical trial data, which is collected under controlled conditions, RWD offers insights from various sources, including electronic health records, insurance claims, patient registries, wearable devices, and more. This data reflects the everyday experiences of patients and provides a comprehensive view of how treatments work in real-world settings. Notable Labs uses advanced analytics, including big data analytics, machine learning, and artificial intelligence, to integrate and analyze this data, uncovering patterns and generating insights that enhance the precision and effectiveness of medical interventions.
Through the use of RWD, Notable Labs is overcoming the limitations of clinical trial data, such as limited generalizability and a lack of long-term follow-up. By broadening patient representation and monitoring real-world effectiveness and safety, they support the development of personalized treatment protocols. Notable Labs' innovative approach is paving the way for a new era of healthcare, providing more precise, equitable, and patient-centered care.
Challenges and Considerations in Using Real-World Data
While the benefits of RWD in precision medicine are substantial, there are also significant challenges and considerations to address. One of the primary challenges is ensuring the quality and standardization of data. RWD comes from diverse sources with varying levels of detail, accuracy, and consistency. Data quality issues such as missing data, coding errors, and variations in data collection practices can introduce biases and affect the reliability of analyses. To address these issues, it is crucial to implement robust data quality control measures and standardize data collection and reporting practices across different sources.
Another critical consideration is the ethical and regulatory landscape surrounding the use of RWD. Patient privacy and data protection are paramount concerns, particularly given the sensitive nature of health information. Regulations such as the Health Insurance Portability and Accountability Act (HIPAA) in the United States and the General Data Protection Regulation (GDPR) in Europe impose strict guidelines on the collection, storage, and use of personal health data. Navigating these regulations requires careful planning and adherence to best practices to ensure that patient data is used ethically and responsibly.
The ethical implications of using RWD extend beyond privacy concerns. There are also considerations related to the potential for misuse of data, such as using it to deny insurance coverage or employment opportunities. Additionally, the use of RWD in research and treatment decisions must be guided by principles of fairness and equity, ensuring that all patients have access to the benefits of precision medicine. This includes addressing disparities in data availability and representation, as certain groups may be underrepresented in RWD sources.
Future Directions
Looking ahead, the future of RWD in precision medicine is bright, with numerous opportunities for innovation and improvement. Advances in data collection technologies, such as wearable devices and mobile health applications, are making it easier to collect detailed and continuous data on patients' health and behavior. These technologies, combined with advancements in big data analytics, machine learning, and artificial intelligence, are enabling more sophisticated analyses of RWD, leading to new insights and discoveries.
One promising area of future development is the integration of genomic data with RWD. As the cost of genomic sequencing continues to decrease, it is becoming increasingly feasible to incorporate genetic information into RWD analyses. This integration has the potential to significantly enhance our understanding of the genetic basis of diseases and the role of genetic variability in treatment response, further advancing the goals of precision medicine.
Another important direction is the continued collaboration between stakeholders, including healthcare providers, researchers, regulators, and patients. Effective collaboration is essential for addressing the challenges and maximizing the benefits of RWD. This includes developing standardized data collection and reporting practices, establishing clear ethical guidelines, and creating frameworks for data sharing and collaboration that respect patient privacy and autonomy.
Conclusion
In conclusion, real-world data plays a crucial role in advancing the goals of precision medicine. By providing insights from a diverse and representative patient population, RWD complements clinical trial data and enhances our understanding of the real-world effectiveness and safety of treatments. The integration of RWD with clinical trial data, supported by advanced analytics, is enabling the development of more personalized and effective treatment protocols, improving patient outcomes across a wide range of medical conditions.
However, the use of RWD also presents challenges, including issues related to data quality, standardization, and ethical considerations. Addressing these challenges requires a concerted effort from all stakeholders involved in the healthcare ecosystem. As we look to the future, continued innovation in data collection and analysis, along with collaborative efforts to address ethical and regulatory concerns, will be key to realizing the full potential of RWD in precision medicine.
Ultimately, the goal of precision medicine is to provide more effective, personalized care that improves the quality of life for all patients. By leveraging the power of real-world data, we are taking significant steps toward achieving this goal, paving the way for a new era of healthcare that is more precise, equitable, and patient-centered.
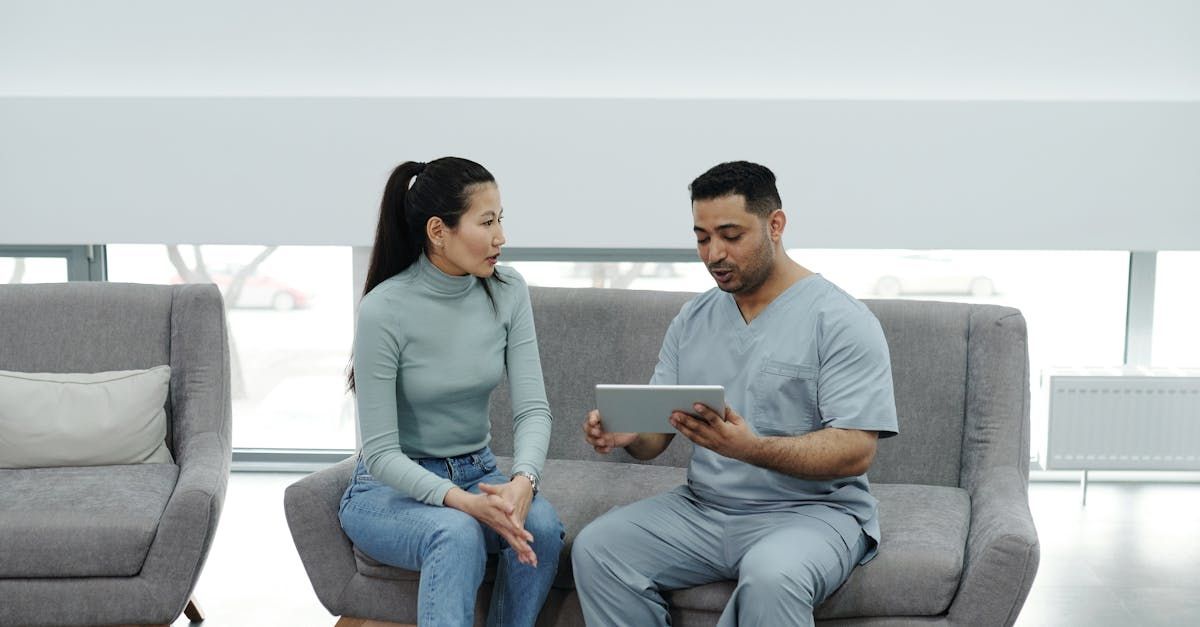
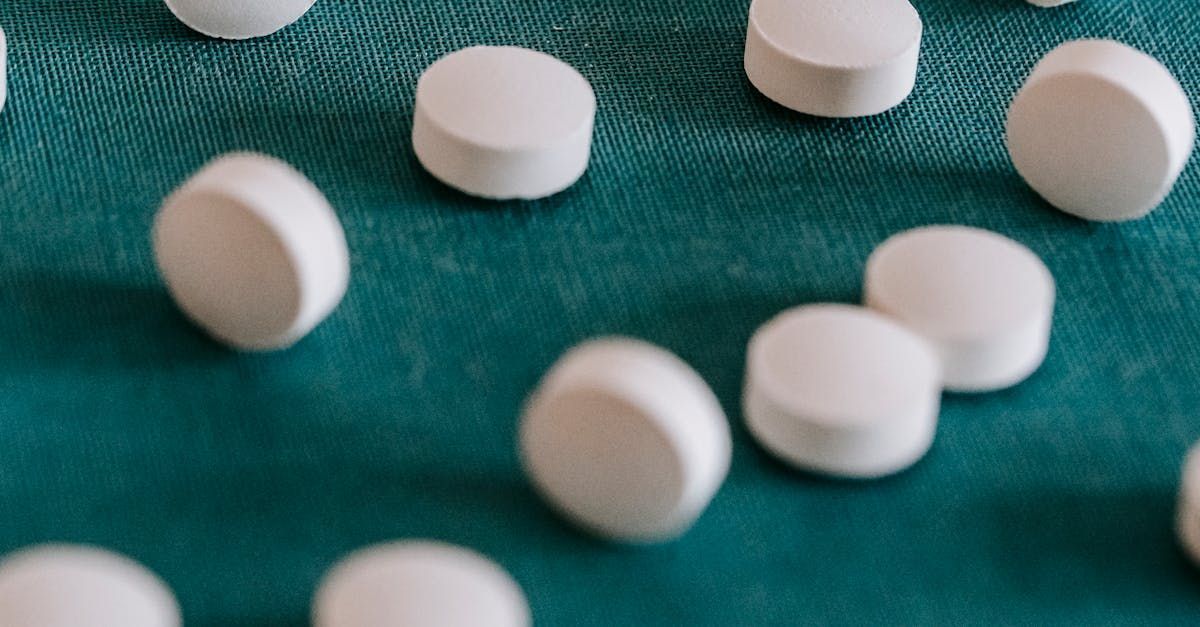
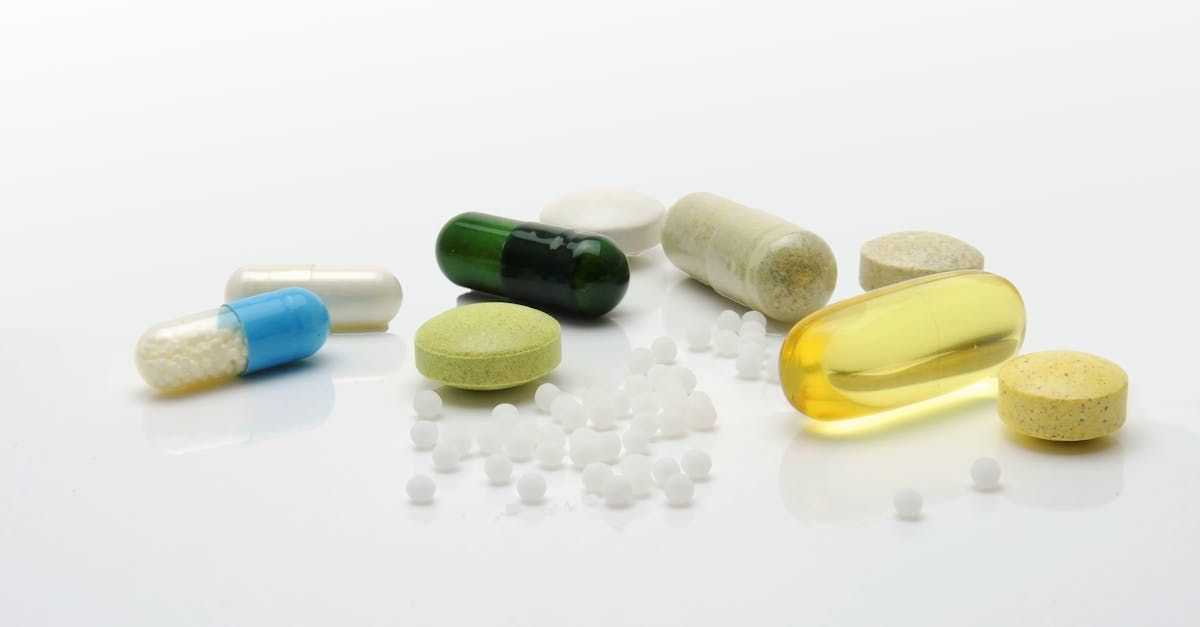
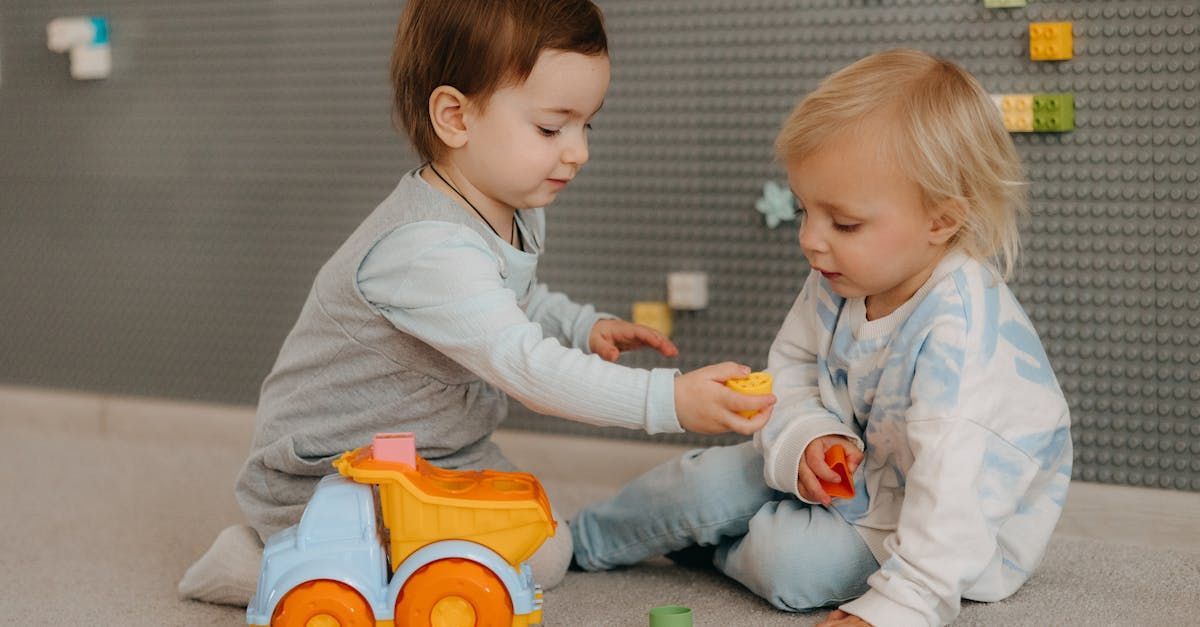
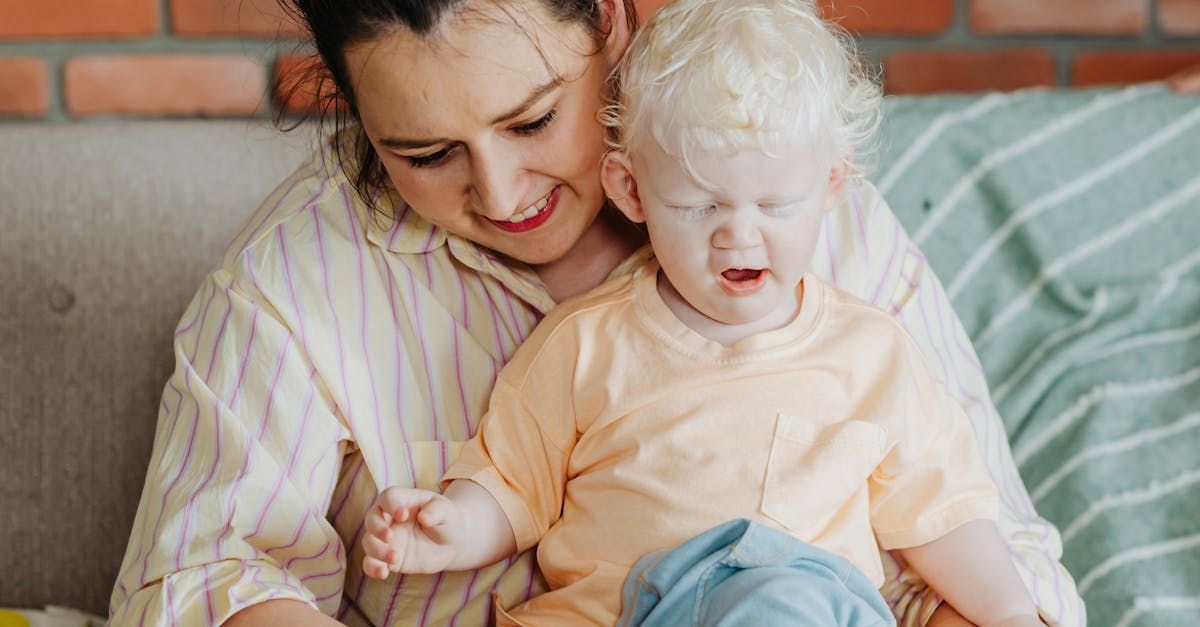
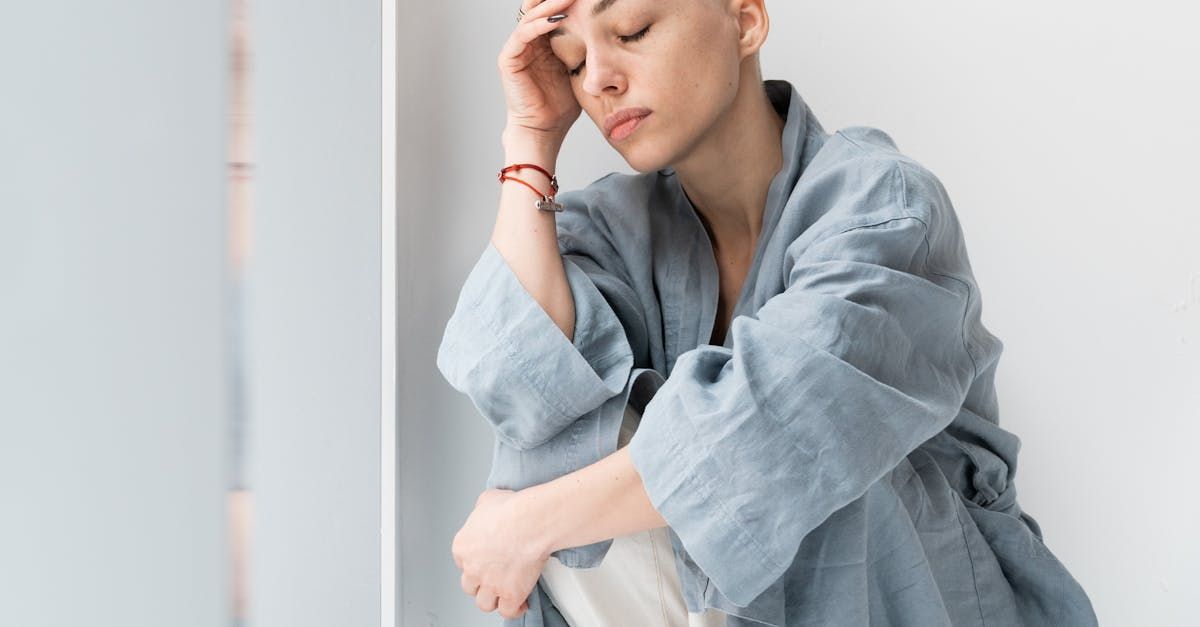
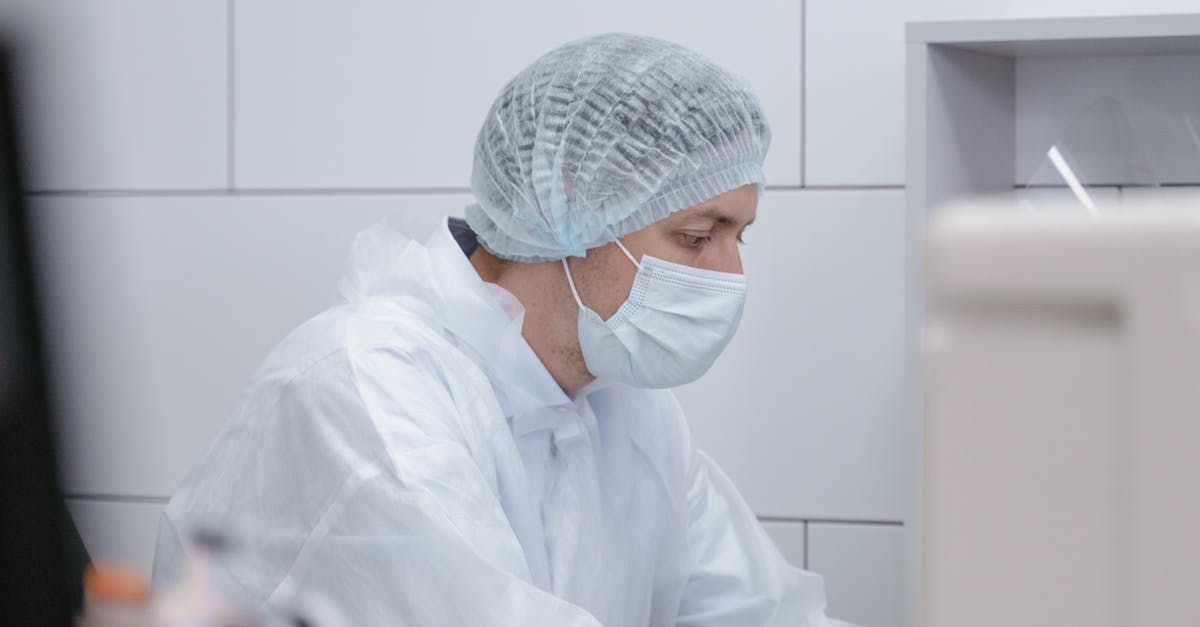
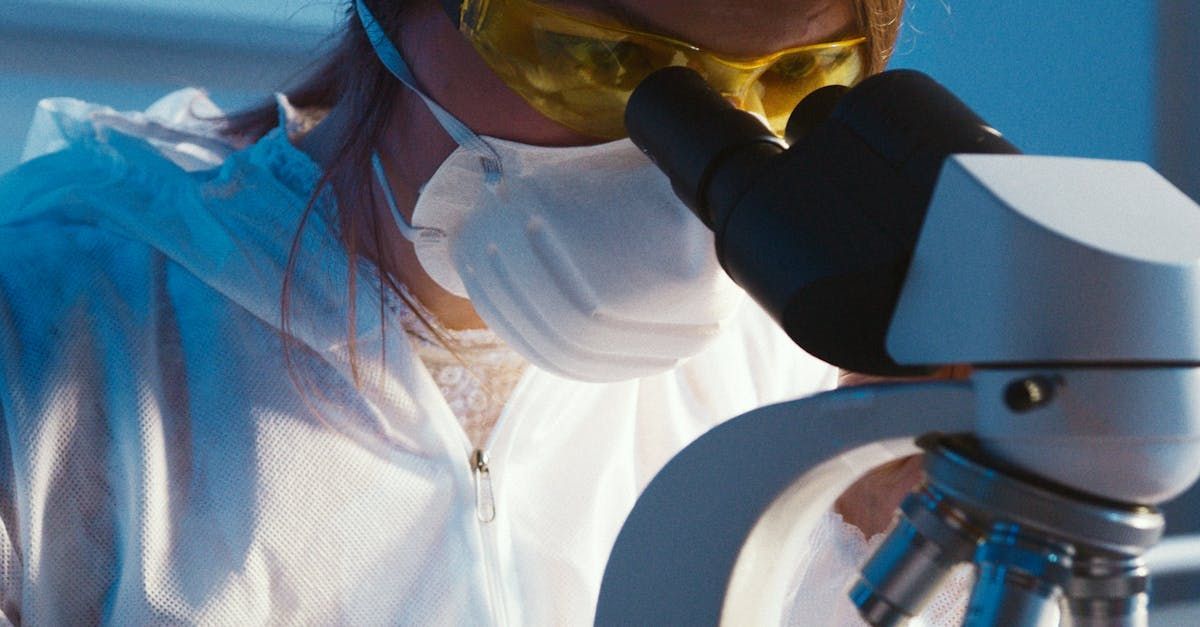
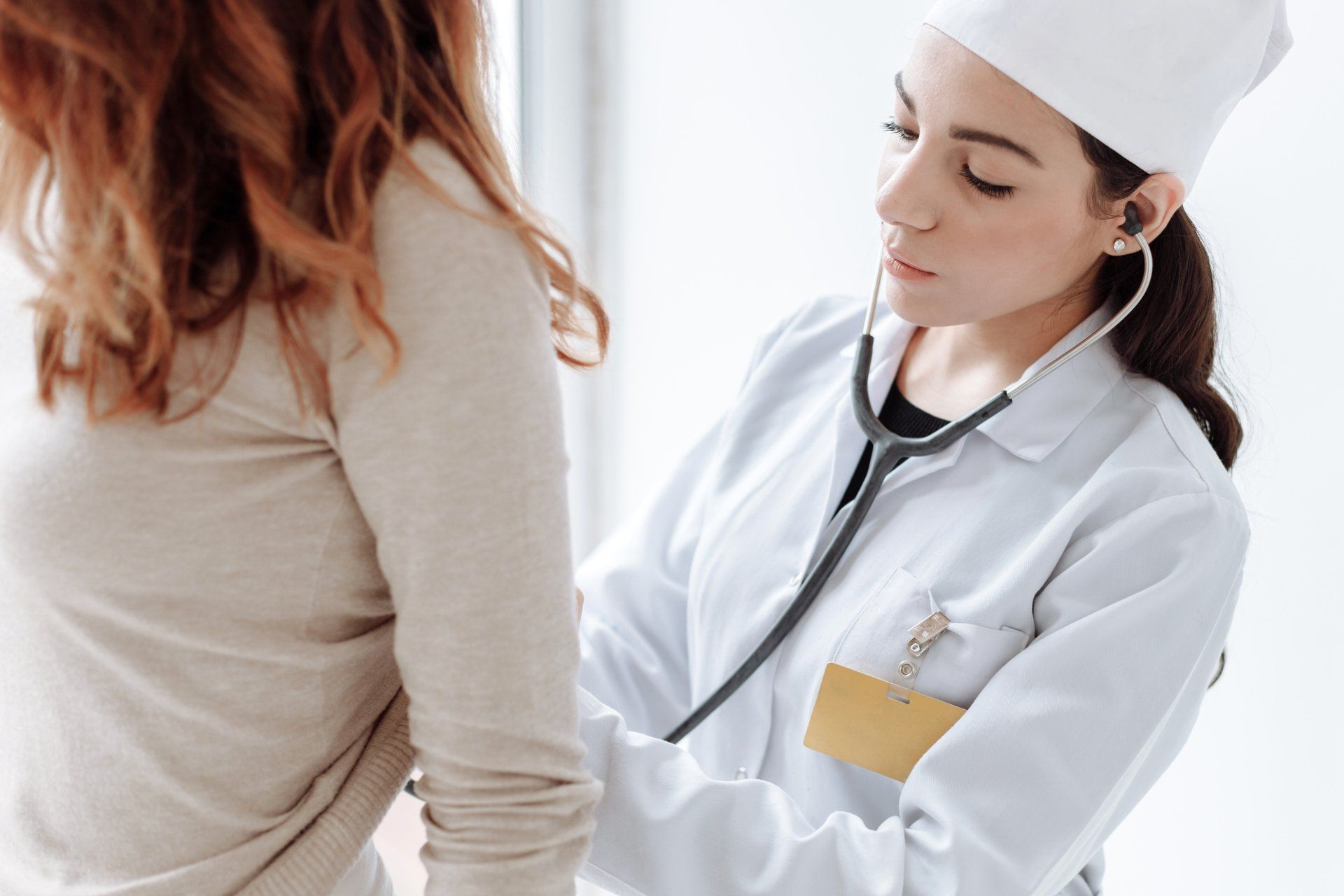
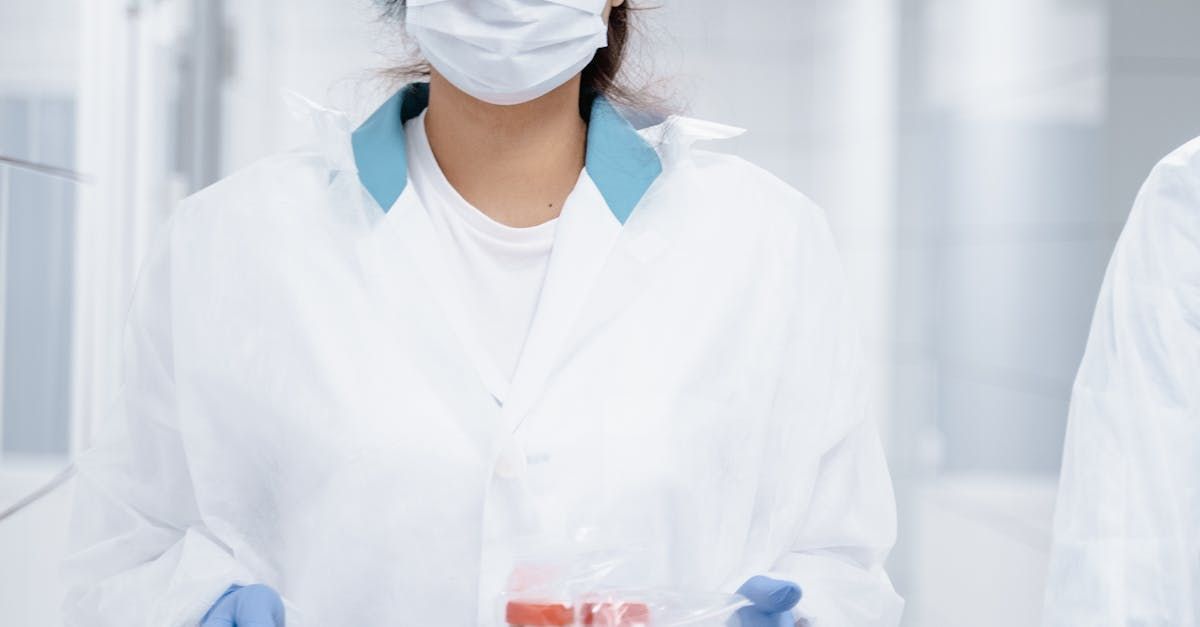