The Use of Big Data in Clinical Trials
In the contemporary era of digital transformation, the concept of "big data" has permeated nearly every industry. Big data refers to the massive volumes of structured and unstructured data generated at unprecedented speeds and in diverse formats. This data explosion, driven by the proliferation of digital technologies, presents both opportunities and challenges. The potential of big data lies in its ability to provide deep insights through advanced analytics, transforming decision-making processes and operational efficiencies across various fields, including finance, marketing, and healthcare. Among these, healthcare stands out as an area where big data can have profound implications, particularly in the realm of clinical trials.
Clinical trials are fundamental to the advancement of medical science. They serve as the primary method for evaluating the safety, efficacy, and effectiveness of new drugs, therapies, or medical devices. The rigorous and systematic nature of clinical trials ensures that new treatments are thoroughly tested before they are made available to the public. Despite their critical role, clinical trials are often plagued by challenges such as high costs, extended timelines, patient recruitment difficulties, and complex data management requirements. Big data analytics offers a promising solution to these challenges by providing a more nuanced and comprehensive approach to managing and analyzing clinical trial data.
The Intersection of Big Data and Clinical Trials
The intersection of big data and clinical trials represents a paradigm shift in how medical research is conducted. Big data analytics involves the use of advanced computational techniques to analyze large and complex data sets. This allows researchers to uncover patterns, correlations, and trends that would be impossible to detect with traditional methods. In the context of clinical trials, big data analytics can be utilized to optimize various aspects of the trial process, from design and patient recruitment to data collection and analysis.
One of the primary benefits of incorporating big data into clinical trials is the enhanced ability to utilize diverse data sources. Clinical trials traditionally rely on data collected during the trial itself, which may not fully capture the variability and complexity of real-world patient populations. Big data allows researchers to integrate information from a variety of sources, including electronic health records (EHRs), genomic data, patient-reported outcomes, social media, and data from wearable devices. This integration enables a more comprehensive understanding of patient demographics, disease mechanisms, and treatment responses, leading to more personalized and effective healthcare solutions.
Big Data's Role in Trial Design
The design phase is one of the most critical stages in the lifecycle of a clinical trial. A well-designed trial not only ensures the scientific validity of the study but also enhances its feasibility and ethical soundness. Big data analytics can play a crucial role in this phase by improving patient recruitment and selection processes. Traditionally, recruiting patients for clinical trials has been a challenging task due to issues like geographical limitations, lack of awareness, and stringent inclusion/exclusion criteria. Big data can alleviate these challenges by providing a more targeted and efficient approach to patient recruitment.
By leveraging data from EHRs, registries, and other databases, researchers can identify potential trial participants based on specific medical criteria, such as disease stage, genetic markers, or comorbidities. This targeted approach not only increases the likelihood of recruiting eligible participants but also ensures a more diverse and representative sample. For example, big data can help identify underrepresented populations, such as ethnic minorities or patients with rare diseases, who are often excluded from clinical trials. This inclusivity is crucial for ensuring that trial results are generalizable to the broader patient population.
Enhanced patient matching is another significant advantage of using big data in clinical trial design. Precision medicine, which tailors treatment to individual patient characteristics, is increasingly becoming a focus in medical research. Big data analytics can facilitate precision medicine by enabling researchers to identify subgroups of patients who are more likely to respond to specific treatments. For instance, by analyzing genetic data, researchers can identify biomarkers that predict a patient's response to a particular drug. This information can then be used to stratify patients into different treatment arms, increasing the trial's efficiency and success rate.
Study design optimization is also a critical area where big data can make a substantial impact. Traditional trial designs often rely on limited data and are based on assumptions that may not accurately reflect real-world conditions. Big data analytics can provide a more nuanced understanding of these conditions, allowing researchers to tailor study protocols more effectively. For example, predictive analytics can help determine the optimal number of participants needed to achieve statistically significant results, minimizing the risk of underpowered studies. Additionally, big data can inform the selection of trial sites by analyzing factors such as disease prevalence, healthcare infrastructure, and patient demographics. This ensures that trials are conducted in the most relevant and accessible locations, enhancing the generalizability and impact of the findings.
Another crucial aspect of trial design where big data can contribute is risk assessment and management. Clinical trials inherently carry various risks, including adverse events, patient non-compliance, and data integrity issues. Big data analytics can help mitigate these risks by providing a more comprehensive risk assessment framework. For instance, historical data from previous trials or clinical practice can be used to identify risk factors associated with specific patient populations or treatment modalities. Machine learning algorithms can also be employed to detect early warning signs of adverse events, enabling timely interventions. By proactively managing risks, researchers can enhance patient safety and improve the overall quality and reliability of the trial outcomes.
Enhancing Data Collection and Analysis
Data collection and analysis are fundamental components of any clinical trial. Traditionally, these processes have been labor-intensive and prone to errors, often relying on manual data entry and periodic data collection. Big data analytics offers a transformative approach to these challenges by enabling real-time data monitoring and analysis. This capability is particularly valuable in adaptive trial designs, where data-driven modifications can be made during the course of the trial to improve outcomes.
Real-time data monitoring involves the continuous collection and analysis of data from various sources, such as electronic health records, wearable devices, and mobile health applications. This approach allows researchers to track patient outcomes and adverse events as they occur, facilitating timely decision-making and interventions. For example, in a trial testing a new cardiovascular drug, wearable devices can continuously monitor patients' heart rates and blood pressure, providing real-time data on the drug's efficacy and safety. This not only enhances the precision of the trial but also reduces the burden on participants, as they do not need to make frequent visits to the study site.
The integration of real-world evidence (RWE) is another significant advantage of big data in clinical trials. RWE refers to data derived from sources other than traditional clinical trials, such as electronic health records, insurance claims, and patient registries. Incorporating RWE into clinical trials provides a more comprehensive understanding of how treatments perform in real-world settings, beyond the controlled environment of a clinical trial. This is particularly important for assessing the long-term safety and effectiveness of new therapies, as well as for understanding how treatments work across diverse patient populations.
Data integration and harmonization are critical challenges in the use of big data for clinical trials. Given the diverse nature of data sources, such as EHRs, genomics, and patient-reported outcomes, it is essential to create a unified and standardized dataset for analysis. This process involves several steps, including data extraction, transformation, and loading (ETL), as well as data cleaning and quality assurance. The goal is to ensure that data from different sources are compatible and can be analyzed together meaningfully.
Machine learning and artificial intelligence (AI) play a vital role in data integration and harmonization. These technologies can automate many of the complex and time-consuming tasks involved in data processing, such as identifying and resolving discrepancies, imputing missing values, and standardizing data formats. For instance, natural language processing (NLP) algorithms can extract relevant information from unstructured data, such as clinical notes or patient narratives, and convert it into structured data for analysis. By leveraging these advanced technologies, researchers can ensure that the integrated dataset is of high quality and suitable for complex analyses, such as predictive modeling and machine learning.
Improving Outcomes and Efficiency
The ultimate goal of integrating big data into clinical trials is to improve outcomes and efficiency. One of the most significant benefits is the acceleration of drug development. Traditionally, the process of bringing a new drug to market can take over a decade and cost billions of dollars. This lengthy timeline is due to the complex and resource-intensive nature of clinical trials, which involve multiple phases of testing, data analysis, and regulatory approval. Big data analytics can streamline this process by optimizing various stages of the drug development pipeline.
For instance, in the early stages of drug discovery, big data can be used to identify promising drug candidates more quickly. By analyzing large datasets of molecular structures, genetic information, and clinical outcomes, researchers can identify potential targets for new drugs and predict their efficacy and safety. This data-driven approach reduces the need for trial-and-error testing, speeding up the discovery process and increasing the likelihood of success.
In the clinical testing phase, big data can enhance the efficiency and effectiveness of trials. Adaptive trial designs, which use real-time data to adjust the trial's parameters, can significantly reduce the time and cost of conducting trials. For example, if interim data analysis indicates that a particular dose of a drug is more effective, the trial can be modified to focus on that dose, reducing the number of participants and the duration of the trial. Additionally, big data can be used to identify biomarkers that predict patient responses to treatment, enabling the stratification of patients into different subgroups. This precision medicine approach not only improves the likelihood of trial success but also ensures that patients receive the most appropriate and effective treatment.
Cost reduction is another significant benefit of big data in clinical trials. By optimizing trial design, improving patient recruitment, and enhancing data management, researchers can reduce the overall cost of conducting trials. For example, using big data to identify the most suitable trial sites can reduce logistical costs, such as travel and accommodation for participants and staff. Additionally, the use of digital health technologies, such as wearable devices and mobile health applications, can reduce the need for in-person visits and laboratory tests, further reducing costs.
Enhanced patient safety and compliance are also critical outcomes of big data in clinical trials. Real-time monitoring and data analytics enable researchers to detect and address safety issues more quickly, minimizing the risk of adverse events. For example, machine learning algorithms can analyze real-time data from wearable devices to detect early signs of adverse reactions, allowing for timely interventions. Additionally, big data can help ensure that patients adhere to trial protocols by tracking their behaviors and providing timely reminders. This is particularly important in long-term studies where patient compliance can be a challenge.
Challenges and Considerations
While the benefits of big data in clinical trials are clear, there are also significant challenges and considerations that must be addressed. One of the most pressing concerns is data privacy and security. Clinical trials involve the collection of sensitive patient data, including medical histories, genetic information, and personal identifiers. Ensuring the protection of this data is paramount, as breaches can lead to serious consequences for patients and institutions. Compliance with regulations such as the General Data Protection Regulation (GDPR) and the Health Insurance Portability and Accountability Act (HIPAA) is essential to safeguard patient privacy and maintain trust.
Data quality and standardization are other critical challenges in the use of big data for clinical trials. The quality of the data used directly impacts the reliability of the findings. Issues such as incomplete data, inconsistent data formats, and errors can compromise the integrity of the analysis. Standardizing data collection methods and implementing rigorous quality control measures are essential to address these challenges. Additionally, the integration of data from diverse sources, such as EHRs, wearables, and genomic data, requires careful consideration to ensure consistency and comparability.
Ethical considerations also play a significant role in the use of big data in clinical research. The use of big data raises questions about consent, transparency, and fairness. For example, patients may not fully understand how their data will be used, and there may be concerns about the potential for data to be used in ways that could harm them. Ensuring that patients provide informed consent and that data use is transparent and equitable is essential to address these ethical concerns. Additionally, researchers must be mindful of biases in data collection and analysis, which can lead to disparities in treatment outcomes.
Notable Labs: Revolutionizing Clinical Trials with Big Data
In the contemporary era of digital transformation, the concept of "big data" has permeated nearly every industry, including healthcare. Big data refers to the massive volumes of structured and unstructured data generated at unprecedented speeds and in diverse formats. This data explosion, driven by the proliferation of digital technologies, presents both opportunities and challenges. The potential of big data lies in its ability to provide deep insights through advanced analytics, transforming decision-making processes and operational efficiencies across various fields. In healthcare, particularly in clinical trials, big data is proving to be a game-changer.
Clinical trials are fundamental to the advancement of medical science. They serve as the primary method for evaluating the safety, efficacy, and effectiveness of new drugs, therapies, or medical devices. Despite their critical role, clinical trials often face challenges such as high costs, extended timelines, patient recruitment difficulties, and complex data management requirements. Big data analytics offers a promising solution to these challenges by providing a more nuanced and comprehensive approach to managing and analyzing clinical trial data.
Notable Labs leverages the power of big data to revolutionize clinical trials. They integrate advanced computational techniques to analyze large and complex data sets, uncovering patterns, correlations, and trends that would be impossible to detect with traditional methods. By utilizing diverse data sources, including electronic health records (EHRs), genomic data, patient-reported outcomes, and data from wearable devices, they provide a more comprehensive understanding of patient demographics, disease mechanisms, and treatment responses. This integration leads to more personalized and effective healthcare solutions.
One of the key areas where Notable Labs excels is in optimizing clinical trial design. By leveraging big data, they improve patient recruitment and selection processes, ensuring a more diverse and representative sample. Their data-driven approach enhances patient matching, enabling precision medicine that tailors treatment to individual patient characteristics. Additionally, they utilize predictive analytics to optimize study design, from determining the optimal number of participants to selecting the most relevant trial sites.
Notable Labs also addresses the critical challenges of data integration and harmonization in clinical trials. Their use of machine learning and artificial intelligence (AI) automates the complex tasks involved in data processing, ensuring high-quality and standardized data sets for analysis. This allows them to provide real-time data monitoring and analysis, facilitating adaptive trial designs and enhancing patient safety.
As they look to the future, Notable Labs is committed to continuing to innovate in the use of big data in clinical trials. They believe that the integration of real-world evidence (RWE) and the growing use of AI and machine learning for predictive analytics will further enhance the efficiency and effectiveness of clinical research. Their goal is to accelerate drug development, reduce costs, and ultimately improve patient outcomes.
Notable Labs is at the forefront of the digital transformation in healthcare, harnessing the power of big data to advance medical research and provide better care for patients.
Conclusion
The future of big data in clinical trials is promising, with emerging trends and technologies poised to further enhance the field. For instance, the use of AI and machine learning for predictive analytics and personalized medicine is expected to grow. These technologies have the potential to revolutionize clinical trials by providing more accurate predictions of treatment outcomes, identifying new therapeutic targets, and enabling more personalized and effective treatments.
Another emerging trend is the increased use of real-world evidence (RWE) in clinical trials. As healthcare systems increasingly adopt digital technologies, the availability of RWE is expanding. This data provides valuable insights into how treatments perform in real-world settings, beyond the controlled environment of clinical trials. Incorporating RWE into clinical trials can enhance the external validity of the findings and provide a more comprehensive understanding of treatment outcomes.
To fully realize the potential of big data in clinical trials, it is essential for stakeholders to embrace these technologies and invest in the necessary infrastructure. This includes developing robust data management systems, training researchers and clinicians in data analytics, and fostering collaboration across institutions and industries. By doing so, we can overcome the challenges and unlock the full potential of big data to improve clinical trial outcomes and ultimately enhance patient care.
In conclusion, the integration of big data into clinical trials represents a significant advancement in medical research. By leveraging data-driven insights, researchers can enhance trial design, improve patient recruitment and selection, optimize study protocols, and ensure patient safety. While challenges such as data privacy, quality, and ethical considerations must be addressed, the benefits of big data in clinical trials are clear. As the field continues to evolve, big data will play an increasingly important role in advancing medical knowledge and improving patient outcomes. This transformative potential underscores the importance of continued investment in technology and data infrastructure, as well as the need for collaboration and innovation in the healthcare sector.
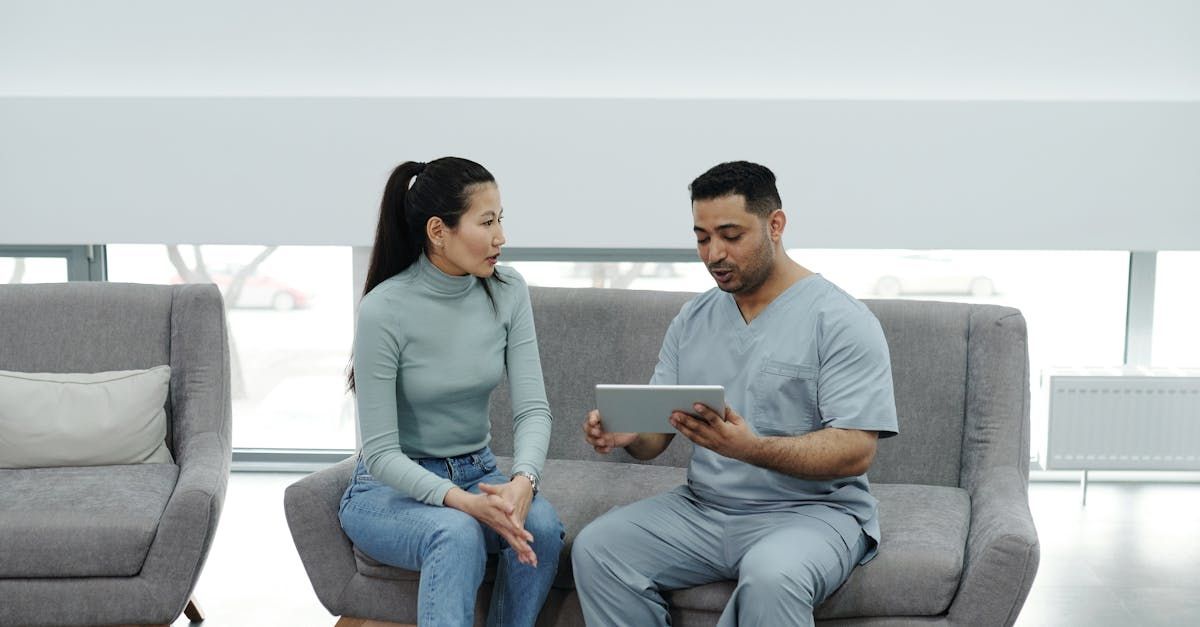
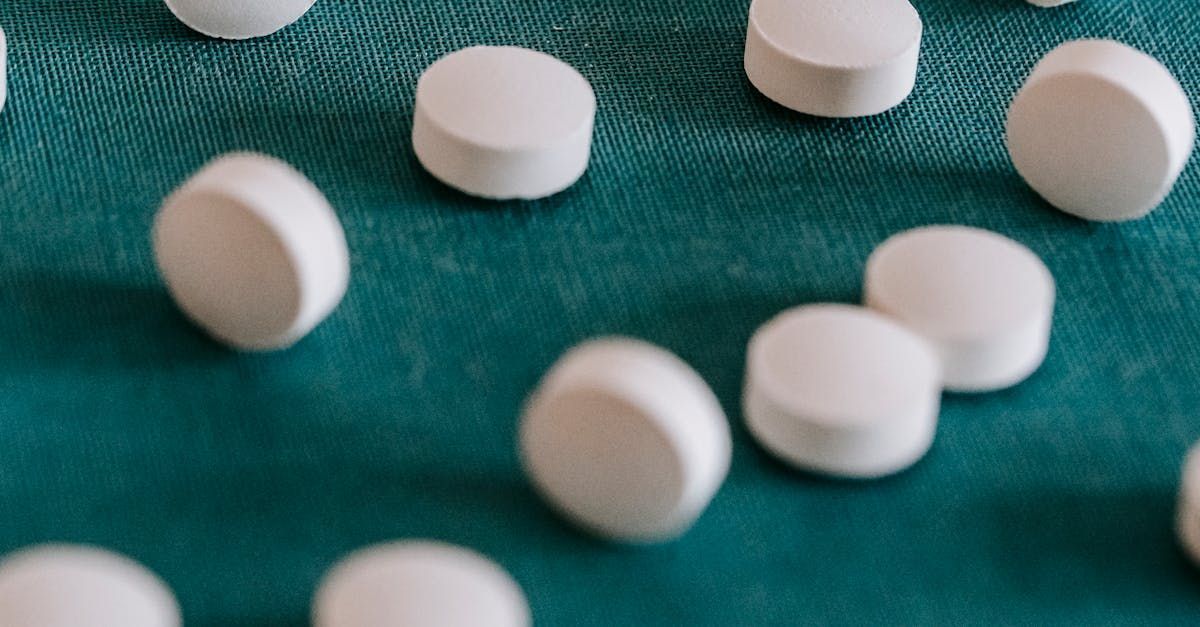
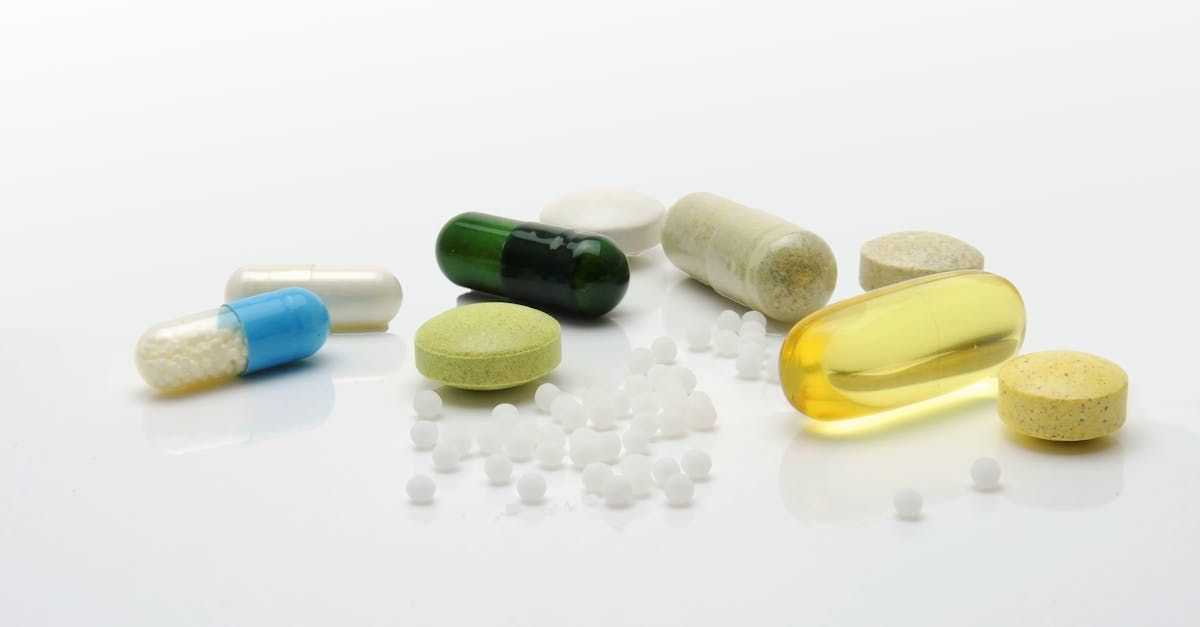
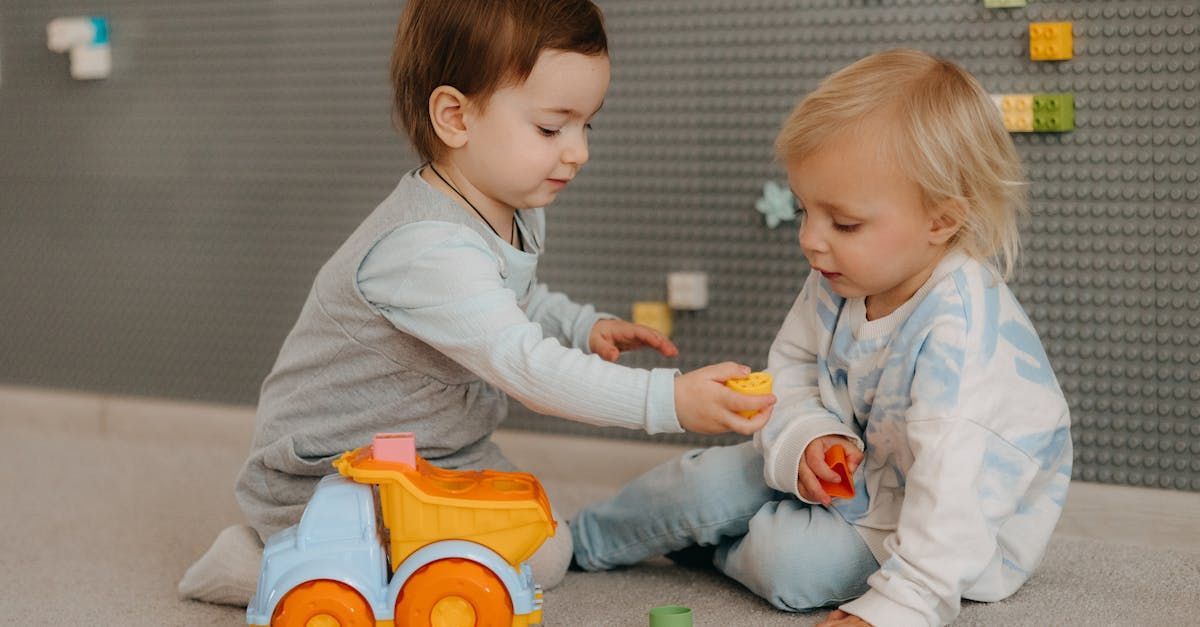
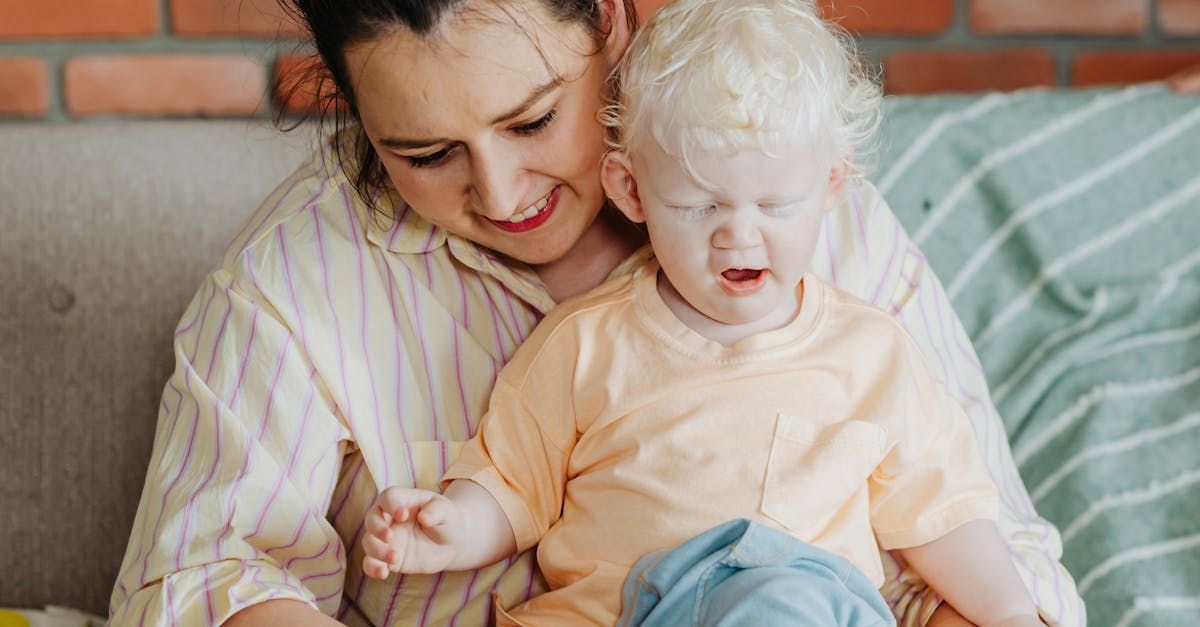
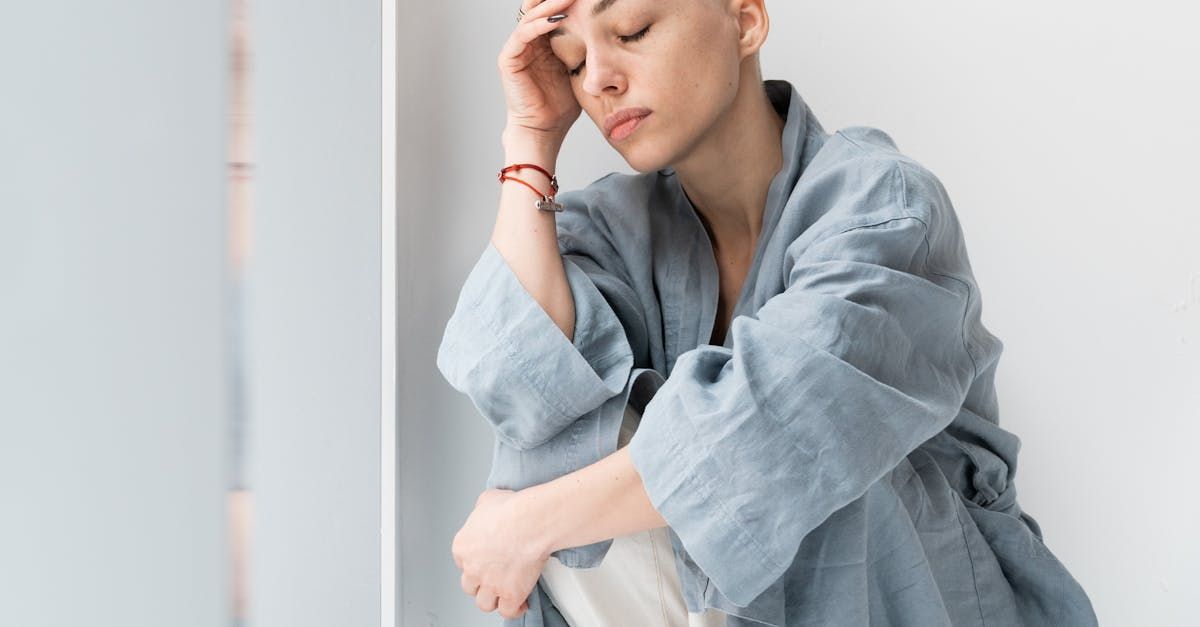
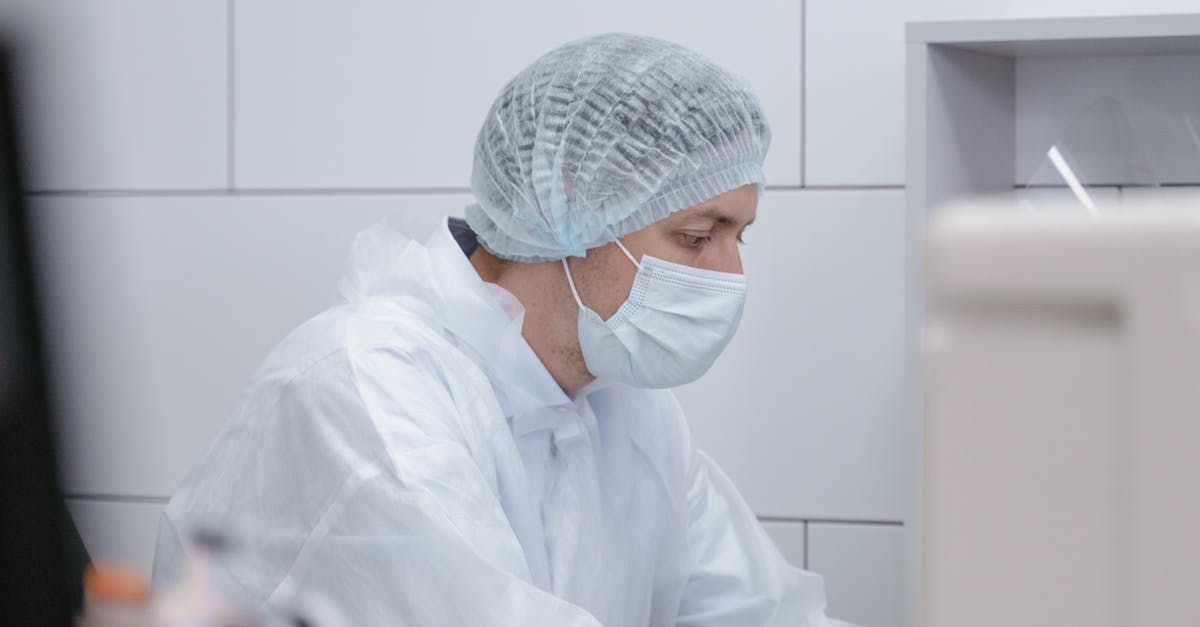
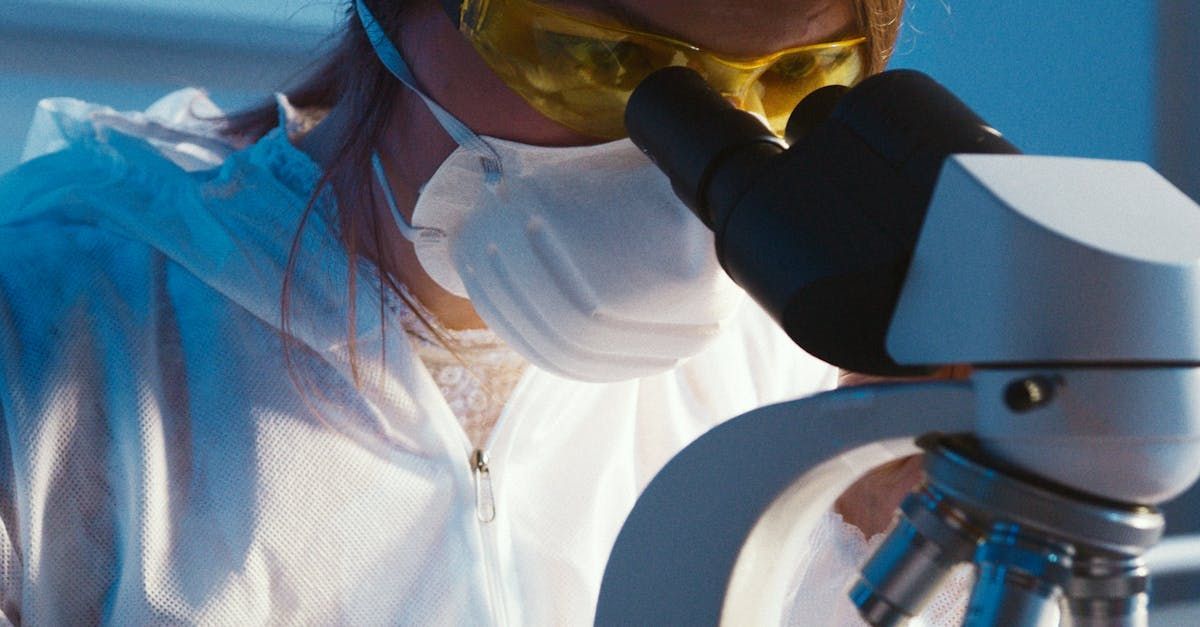
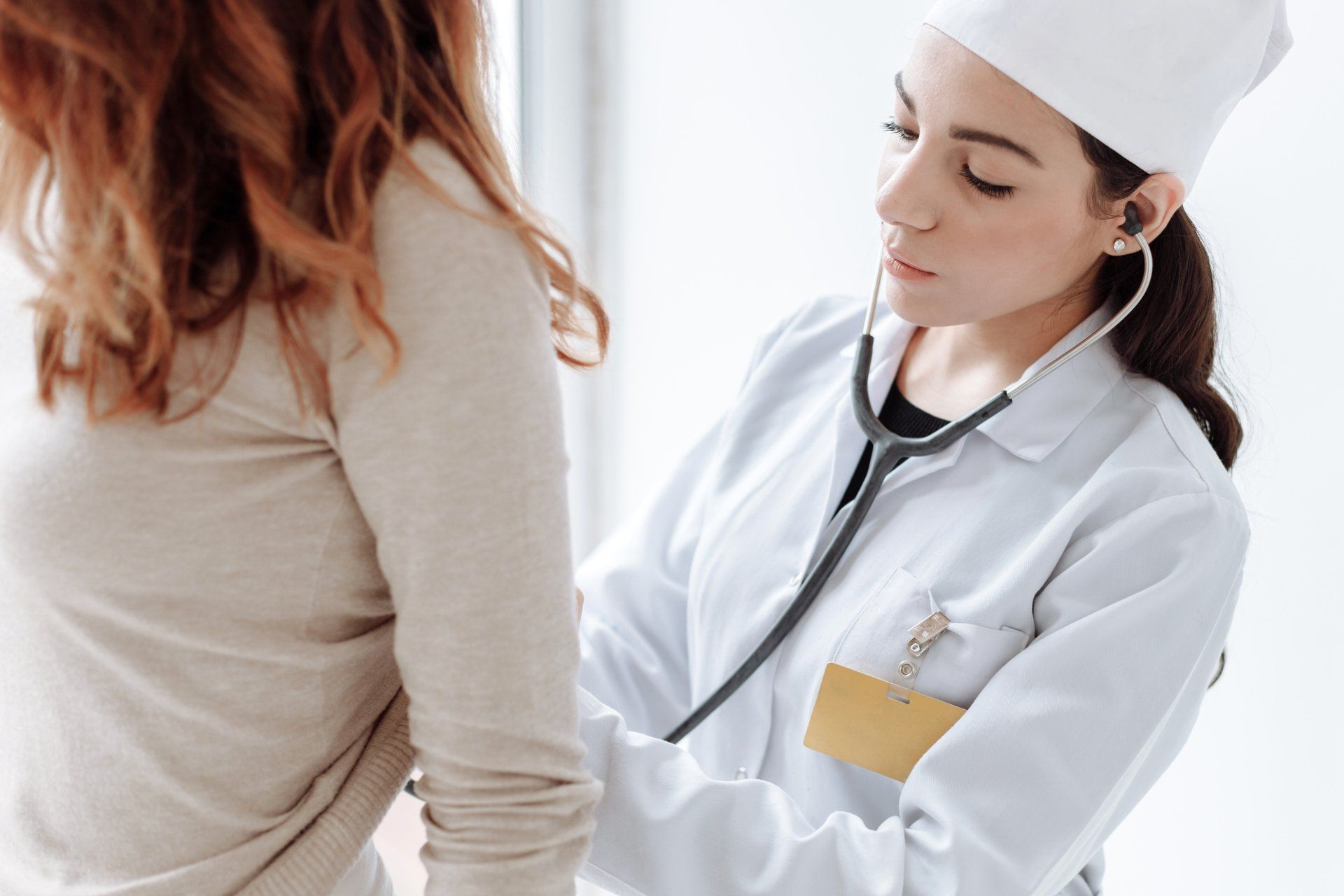
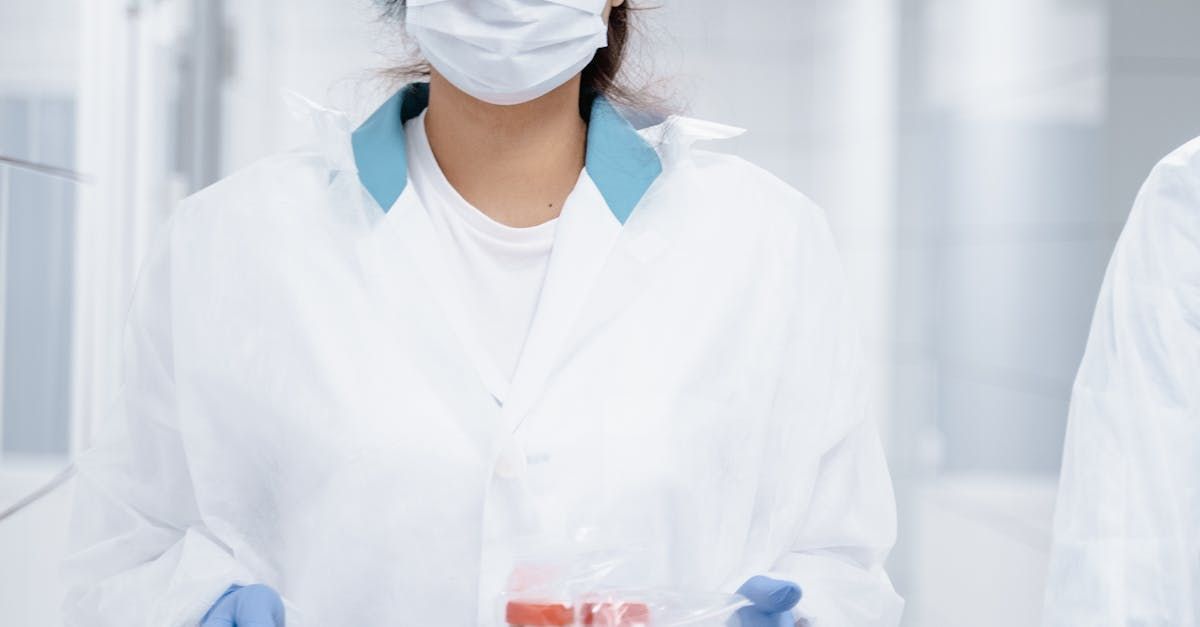